What Is Multimodal AI?
It is a machine learning that merges data from various methosd to train models that can better know the world. These methods could be visual, audio, or textual, and merging them can enhance the details and reliability of AI systems. Multimodal AI works by merging details from each modality and sustaining it into a single model, which can then use these details to make forecasts.
What Is Rare About Multimodal AI?
Multimodal models describe an essential advancement in the platform of artificial intelligence. These machine learning models, also termed multimodal deep learning models, have attained immense popularity, and are identified due to their capability to procedures and knowledge from various methods or sources. They are just starting to combine but are already getting a lot of awareness and showing hopeful advancements in how we cooperate with smart systems.
The mixture procedures are frequently succussed through complicated neural network structures, with the converted model being an important choice. The outcomes are a model that can detain complex relationships and dependencies via various types of data.
How Multimodal Models Work?
Multimodal AI systems can knowledge of many distinct types of data, such as words, pictures, sounds, and video. To make an impression of all this data, the multimodal AI deploys various unimodal neural networks for each data type. So, there’s a component that great at knowledge pictures and another part that’s great at understanding words.
These neural networks pass essential features from the input data. They are frequently manufactured using three main modules that work in combination to allow the model to procedures and knowledge details from diversified methods.
Applications Of Multimodal AI
Image Caption Generation
Multimodal AI systems are pre-owned to simultaneously create graphic captions for images, making content more details and available.
Video Analysis
They are deployed in video analysis, merging visual and auditory data to identify activities and incidents in videos.
Speech Identification
Multimodal AI, like OpenAI’s, is employed for speech identification, translating spoken language in audio into plain text.
Content Generation
These systems create content, such as images or text, based on textual or digital inspiration, improving content creation.
Healthcare
Multimodal AI is useful in medical imaging to evaluate complicated datasets, such as CT scans, aiding in disease diagnosis and treatment planning.
Autonomous Driving
Multimodal AI assists autonomous vehicles by proceeding data from multiple sensors and enhancing navigation and safety.
1. Improved Understanding
Multimodal AI can offer a more thorough and slight understanding of data by studying details from various sources. By merging these divergent data points, the model gains a richer context for evaluation. Its context enables the model to know the content from multiple outlooks and consider information that may not be obvious with an unimodal reach.
Multimodality provides the system the capabilities to get what’s going on in a diverse or in the data it’s handling. For instance, if you show a model a picture and some words, it can build out what’s occurring by seeing both the picture and the words jointly. Contextual insights are like providing the algorithm the power to see the big picture, not just the words, and that’s a big contract in making AI more human. In natural language processing, it is critical to correct language eagerness and carry about pertinent responses.
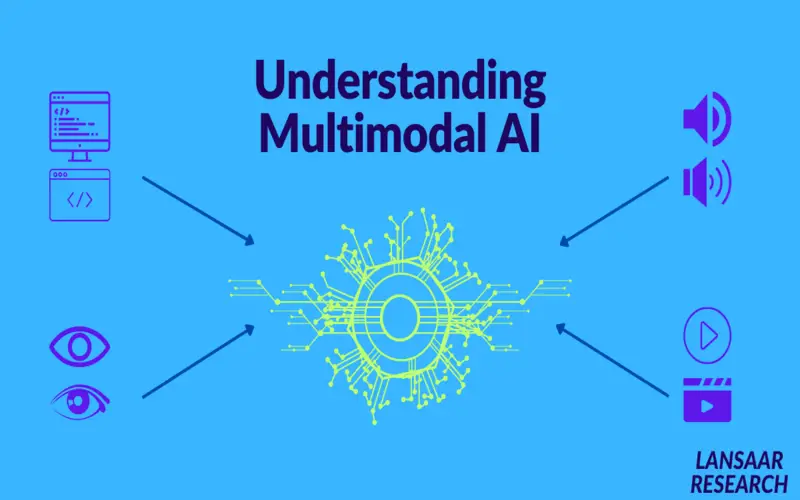
2. Actual-Life Conversations
The assistant sounded robotic and was not very good at insights. That’s because they generally only have insights one way of transmitting, for example just text or speech. Now, multimodal models make it simple for machines to combine with people more naturally. A multimodal virtual assistant, for example, can hear your voice commands, but it also pays awareness to your face and how you are moving. It can recognize your intentions. So, the experience becomes more customized and exciting.
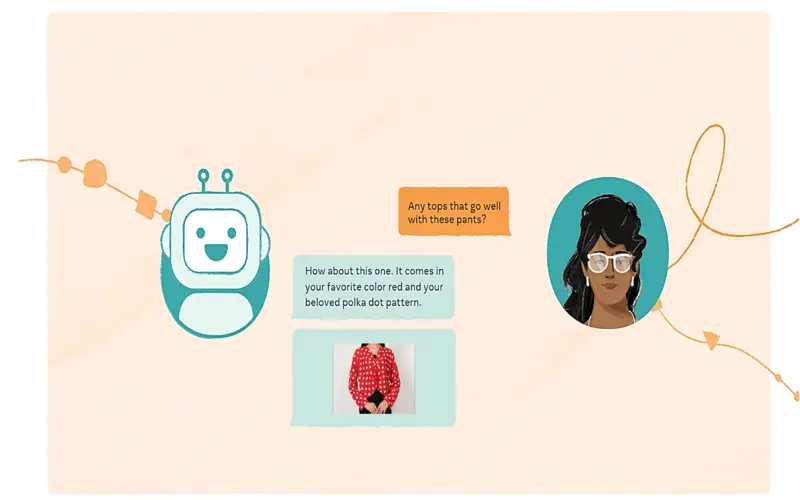
3. Enhanced Accuracy
Multimodal AI models can improve accuracy and minimize issues in their results by carrying details from various methods together. In unimodal AI systems, mistakes can arise from the limitations of a single technique. Multimodal AI can assist in recognizing and accurate errors by combining and approving information across methods. By merging different methods, multimodal AI models can use the strengths of each, leading to a more complete and correct understanding of the data. The occurrence of deep learning and neural networks has played a main role in improving multimodal machine learning accuracy. These models have shown extraordinary abilities in expanding intricate features from information.
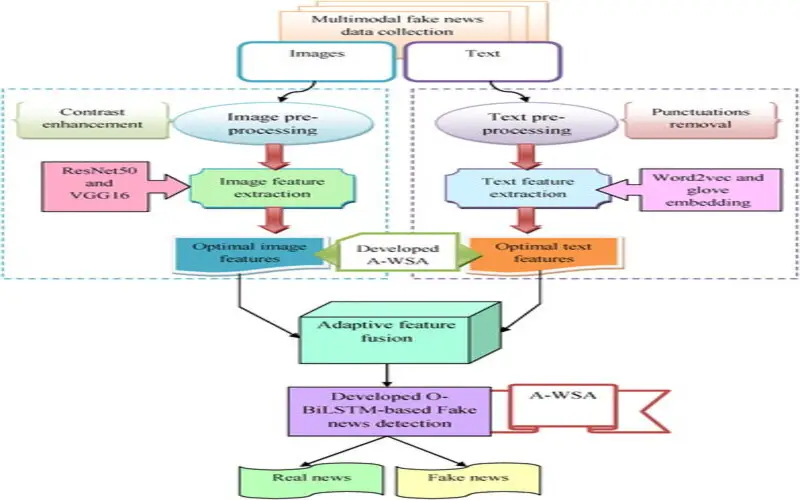
4. Enhanced Capabilities
Multimodal learning for AI/ML extracts the capabilities of the model. A multimodal AI system evaluates many types of data, providing it with a wider insight into the task. It makes the AI/ML model more human-like. For example, a smart assistant trained through multimodal learning can use visual data, pricing information, buying history, and even video data to provide more customized product suggestions.
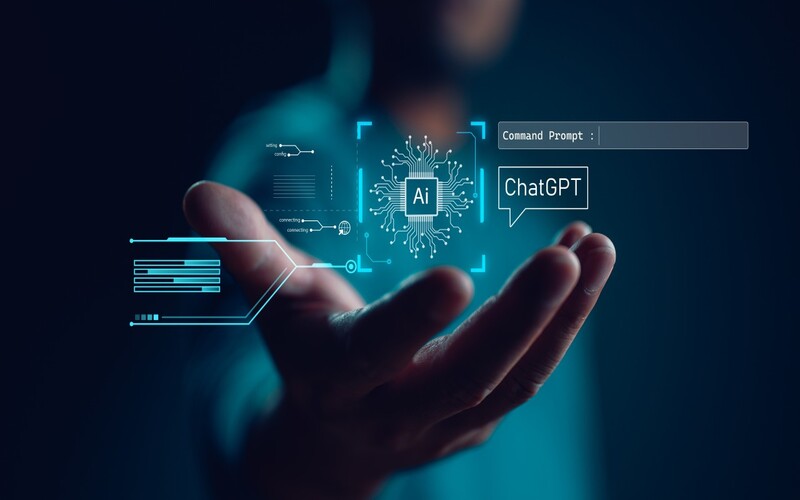
5. Incredible Consumer Experience
It is a virtual assistant that doesn’t just understand your global but also identifies your moves and hears your voice. That’s multimodal AI, creating your interchange continuously and more engaging.
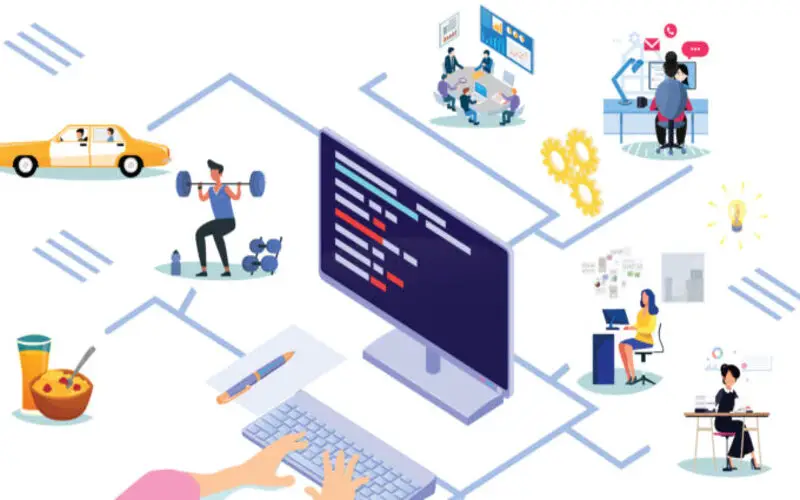
6. Resources Efficiency
Sketch a system sorting through social media posts. Debating not just text but also images and other information can focus on essential ones, saving time and energy.
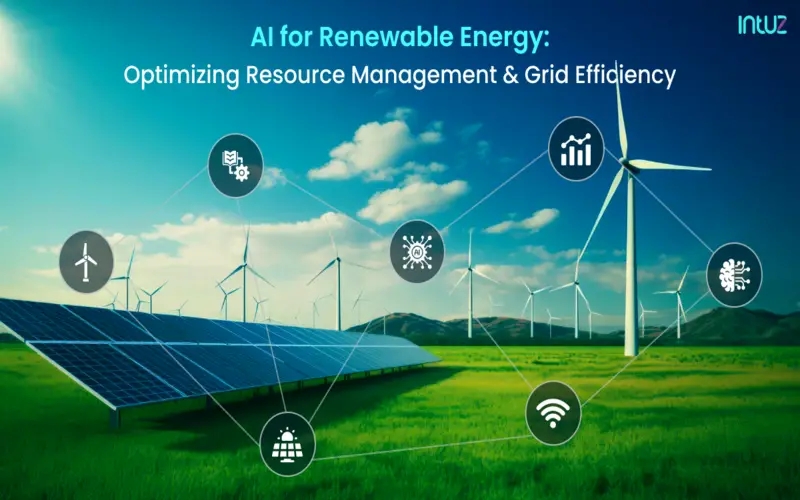
7. Enhanced Interpretation
In the medical treatment system, using not only images but also written discussions assists in explaining why it think’s you are vigor or not. It’s like providing reasons for its decisions, creating, making it more believer.
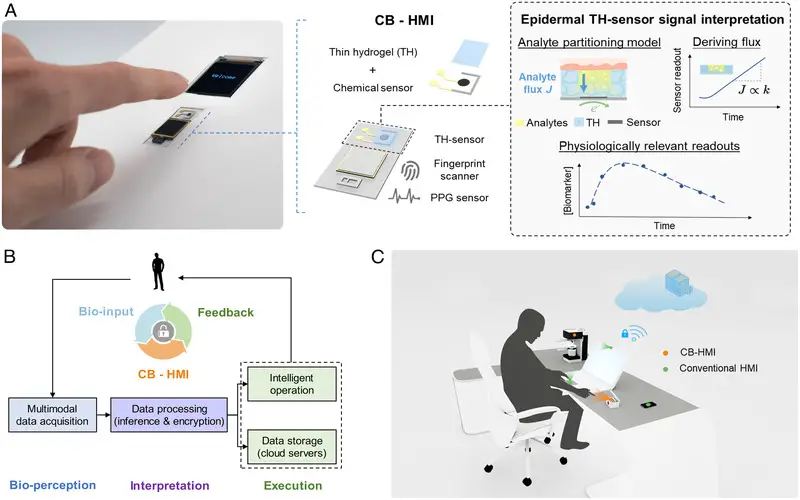
8. Good Natural Language Understanding
A multimodal AI can enhance its capabilities to understand the meaning and purpose behind the words by using other method like facial recognition and accent.
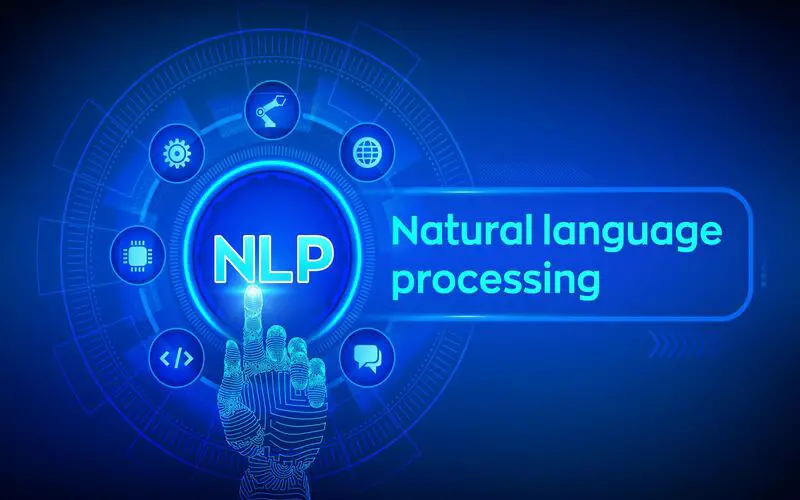
9. Good Human-Computer Interaction
By integrate nonverbal cues such as facial recognition and body language, a multimodal AI can enhance its capabilities with humans more naturally and essentially.
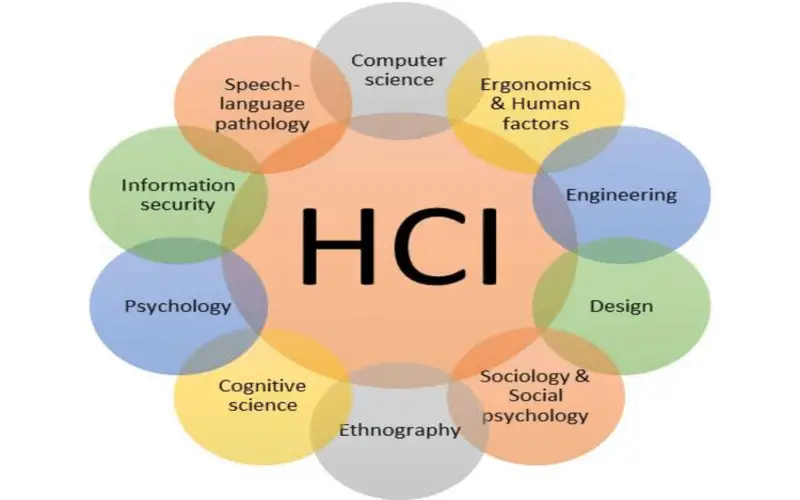
10. Maximized Flexibility
With the capabilities to procedures various forms of data, a multimodal AI can adapt to distinct situations and climates more easily.
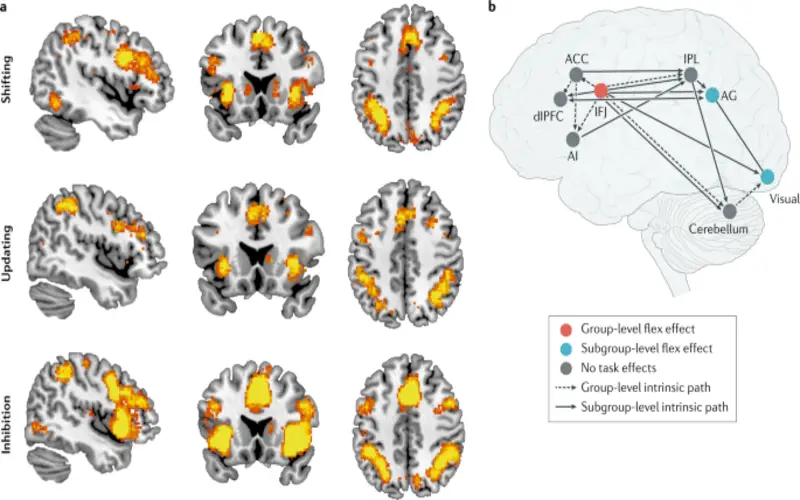
11. Natural Interaction
Another key benefit of multimodal models is their capabilities to advantages natural interaction between humans and machines. Standard AI systems have been limited in collaborate with humans since they commonly depend on a single mode of input, such as text or speech, text, and digital cues, to systemically understand a consumer’s intentions and required.
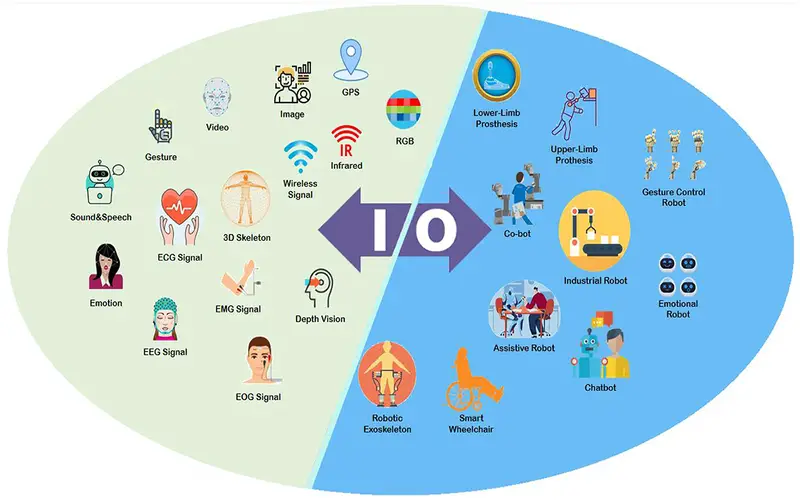