What Are Predictive Analytics?
Advanced analytics includes predictive analytics that estimates future events by combining historical data techniques based on statistical modelling, data mining, and machine learning. The company uses predictive analytics to look for patterns in data to identify dangers and opportunities. Big data and data science are both related to predictive analytics. Predictive Analytics (PA) includes several statistical techniques from Data Mining, Predictive Modelling, Machine Learning, and Deep learning schemes. The central point of PA catches interactions between describing the predicted variables from past episodes and using predictions to known unknown results. The accuracy and effectiveness of the outcomes are significantly relevant to the stage of data analysis and the quality of the statements.
Predictive Analytics Work?
Data scientists use predictive models to specify relationships between diverse elements in selected datasets. The data gathering is over, and a statistical model is now developed and updated to make predictions.
Predictive Analytics Procedures
- The project results, the expansion of the work, and the business goals distinguished the data set usage.
- Data mining for PA makes data from multiple sources for observation and gives a complete view of user interaction.
- Data analysis examines, cleans, and models data to discover usage information for tuning an interference.
- Statistics analysis tests the premise and theory using basic statistical models.
- Predictive modelling automatically generates accurate predictive models about the future.
- The predictive model organization provides the alternative to executing the analytical outcomes in the everyday opinion process.
- Model observing can review the model performance to ensure it provides the expected outcomes.
Features Of Predictive Analytics
1. Data Analysis And Manipulation
Data analysis technology for creating new data sets, altering, clubbing, categorising, combining, and filtering data sets.
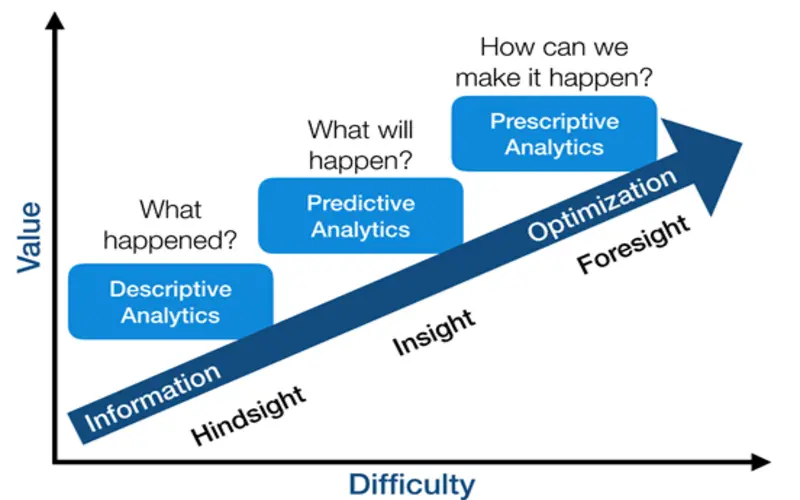
2. Visualization
It includes advantages like graphics and reports that are interactive.
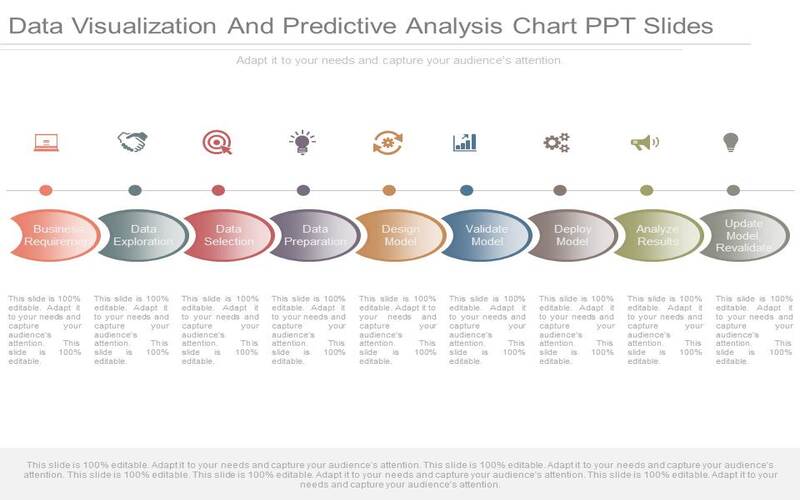
3. Statistics
This technology contributes to the creation and confirmation of links between variables in data. It differs from statistical software and can be integrated with some solutions.
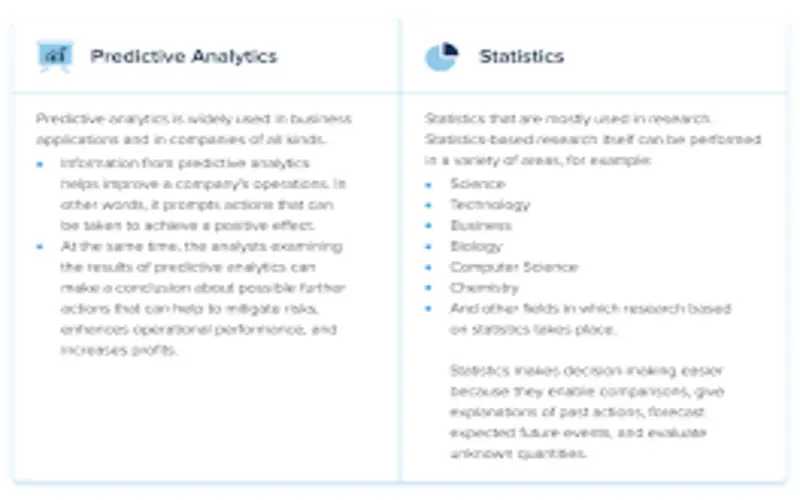
4. Hypothesis Testing
Model creation, evaluation, and selection of the best model for hypothesis testing.
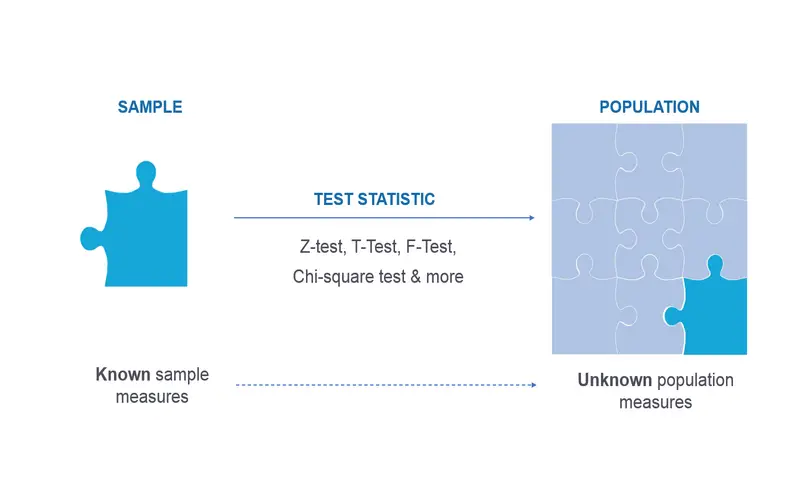
5. Support Automation
It concludes and prevents performance, capacity, availability, and security problems. Capacity management is the most general application of predictive repository software. Models can observe usage per tools, volume, and application in actual time and provide alerts when they hit exertion targets. At the administration’s options, systems can automatically add assigned space or existing capacity to prevent it from running out.
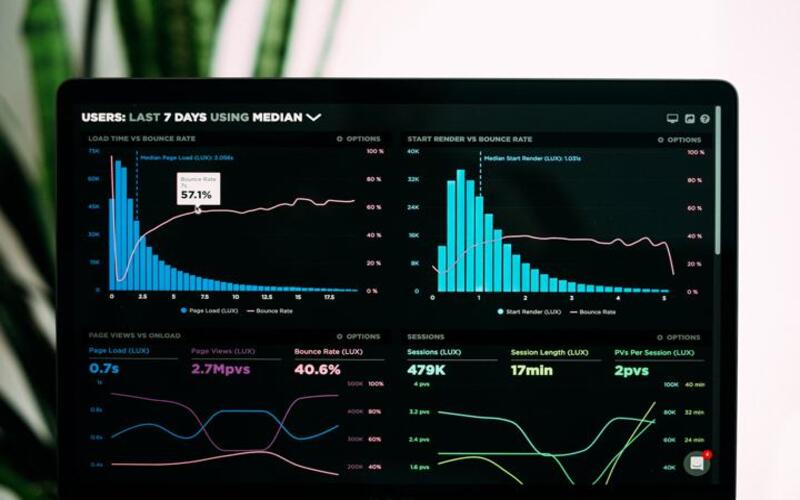
6. Management And Configuration Capabilities
It shows up in models that can immediately determine performance, source consumption, capacity baselines, and trends. It includes ones that don’t have accessible statistical models, such as linear, polynomial reversion, or periodic temporal variations. Improving configuration parameters and collaborating with workload placement, baselines, and trends can automate storage system setup and administration and improve resource consumption and effectiveness.
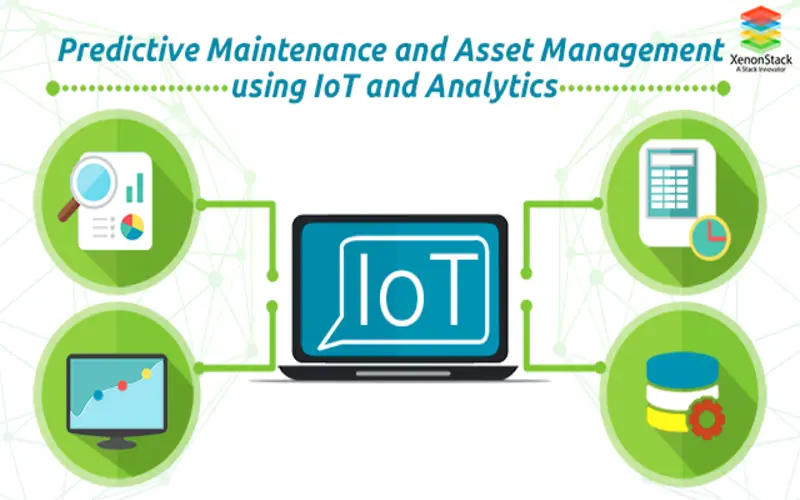
7. System Software Updating And Patching Features
It applies fixes subject to management approval involving hotfixes that address formyl identified system pain. Some retailer uses analytics to make beta or alpha-level code attainable only to sites that experience a single problem and meet hardware or other system requirements in hopes that the update will solve urgent issues without harming the dependability of other consumers.
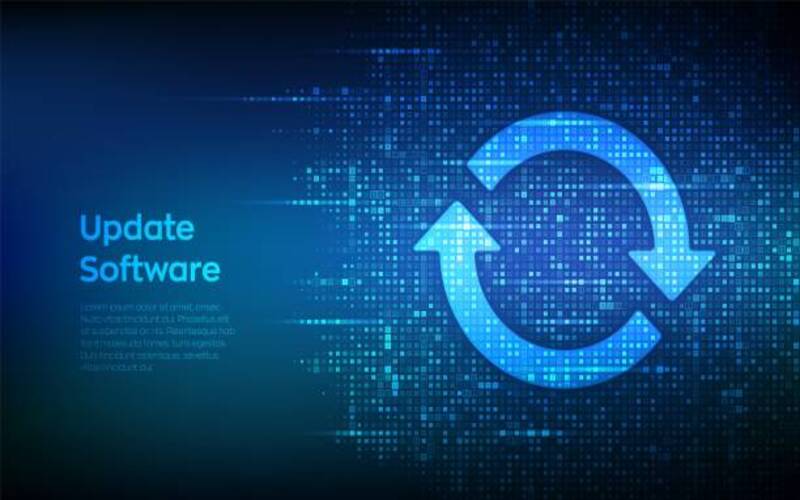
8. Resource Planning Capabilities
The same movement spotting predictive customs to power planning simulations and the analysis that allow repository administrators to rapidly stimulate several schemes and predict capacity, IOPS and bandwidth needs and suggest system upgrades.
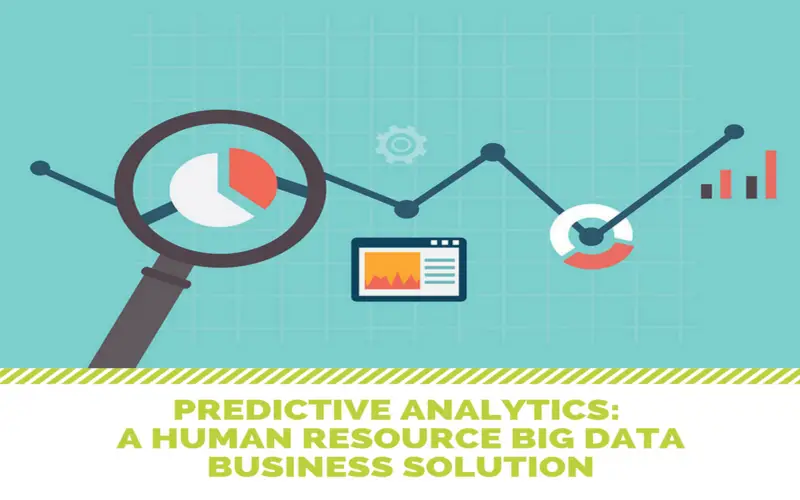
9. Virtual Machine Organizations Feature
Combines with several virtualization platforms notably, VMware’s vSphere to give workload-certain performance and capacity metrics, spot uses tendency and suggest storage configurations settings.
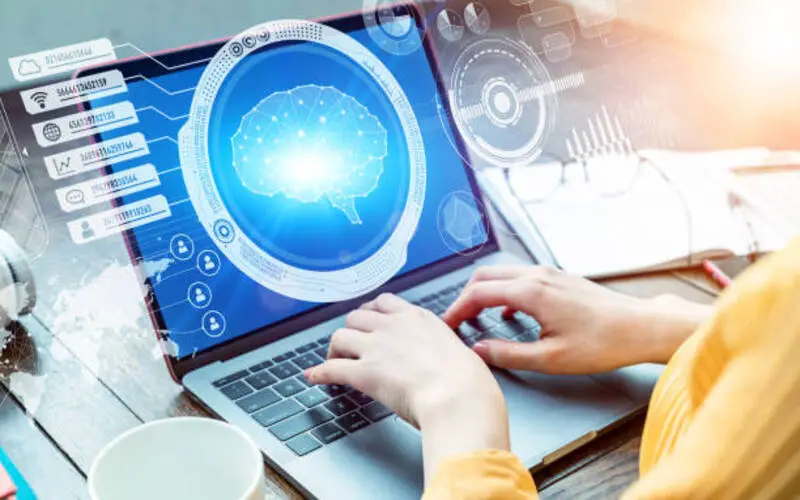
10. Performance Metrics
A well-defined set of performance measures tailored to the management’s business objectives is critical for the effective creation of predictive analytics initiatives.
In the context of various performance indicators, such as R2 or Lift, the model’s growth in predictive analytics figures results in models that appear possible but ultimately fail to perform in the business decision environment.
- The data is divided into categories based on historical knowledge.
- It can be impacted by apps and BI surroundings.
- It organises metric value forecasts by computing new data values using historical data insights.
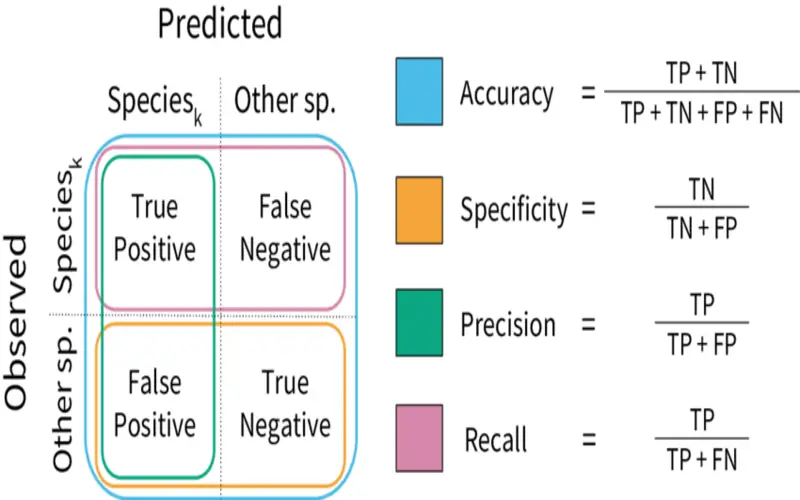
11. Business Features And Integration With SAP Structure
The total integration of the whole predictive analytics procedure with SAP structure. Companies use the application to organize their business application. Many users can use these applications to analyze user behavior and carry out more targeted user marketing. If your business is experiencing high product return rates, using an interaction of PA and SAP HANA can help lower them while allowing you to more accurately target users with offers.
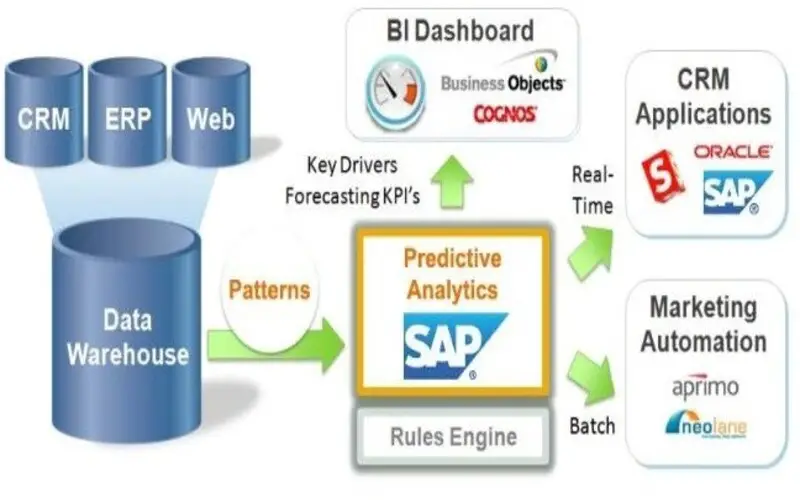