What Is An Autonomous System?
An Autonomous System (AS) in networking is a gathering of one or more connected internet protocol (IP) preface with clearly defined routing protocols that govern how the autonomous system covers routing information with other autonomous systems. An independent system can be considered a connected group of IP networks organized by a single access entry group such as a university, commercial organized, or other type of internet service provider (ISP). The AS distinguishes itself from other network teams in that it must appear to external autonomous systems that it has a single, coherent scatter plan, regardless of how communications occur within the AS.
Challenges And Truth For Building Autonomous Systems
It would be incorrect to suggest that greater use means structuring these systems is easy. It is not because plotting autonomous systems has some unique challenges. Autonomous systems will be used in conditions that were not planned for or predicted; as a result, precision in system requirements will be limited throughout development. The boundary between what a human does and what an autonomous system does during a mission may shift during an operation. As a result, these systems may need energetic functional allocations between humans and machines, and they may be required to learn consistently and take facilities of pattern and open-source parts to improve flexibility and technologies.
1. Loopholes Discovery And Analysis
Maximizing autonomy can enhance efforts in volume, speed, and persistence, especially in observation and reduction. At the same time, the autonomy increases the attack surface and thus the number of loopholes. In addition to typical software and systems loopholes, autonomous systems are at risk from conscious mis-training by attackers, spoofing, and hidden modes. Loopholes in independent control of cyber-physical systems can have more awful outcomes. The increased loopholes of autonomous systems generate a need for consistently red-teaming.
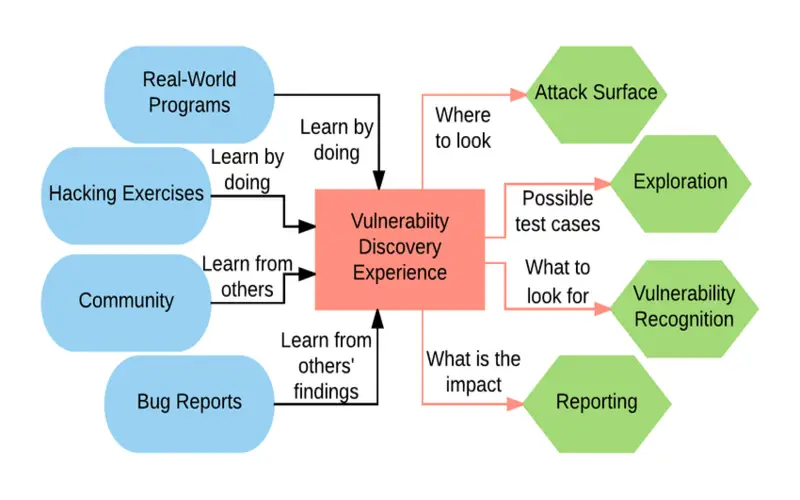
2. Functional Safety Systems
Unsafe autonomous systems are a grave examination of users, governments, and industry watchers. It may seem unfair, but autonomous systems understand a higher standard of safe behaviour than humans. They must demonstrate a higher level of welfare than humans, or they will be rejected by the public. In passenger vehicles, safety features such as lane departure warning and collision detection, as well as active-control systems like as automatic braking and lane-keep assistance, must always function. The public is willing to make mistakes from human drivers.
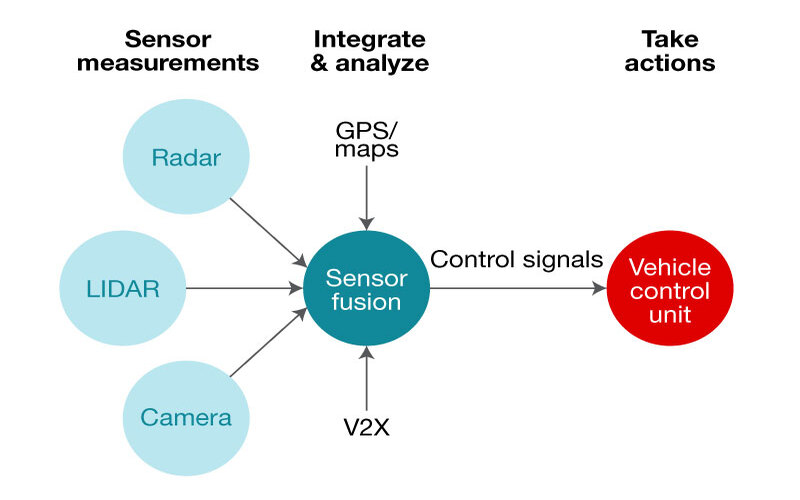
3. Multiple Process Variables
Humans can only consider so many variables. No events how complicated or simple the system is, it is impossible for one person to consider every important factor. Autonomous systems can perform actions that process thousands of variables instantly.
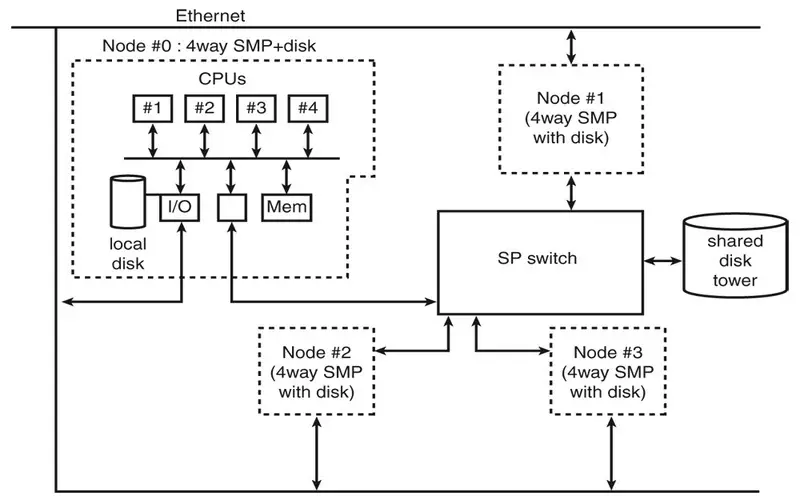
4. Scarcity Of Expertise
Scarcity Of Expertise takes time. As a growing number of factory workers retire, they take with them a wealth of operational knowledge. Inexperience leads to high levels of variation in the final resolution-making. Autonomous systems can help support new employees as they expand the compulsory expertise to proliferate.
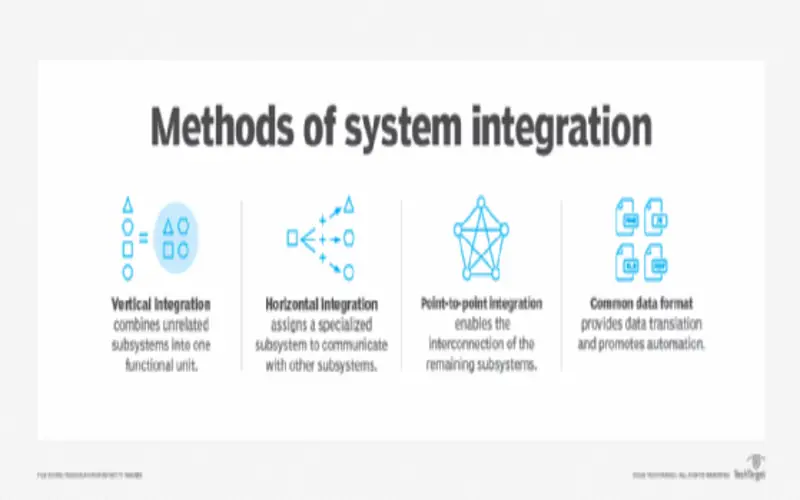
5. Time-Constrained Decisions
Many producing optimization opportunities are short but often. These time-sensitive requests are complicated for human operators to evaluate, organize, and categorize. Autonomous systems can recognize and execute these instantly in actual time.
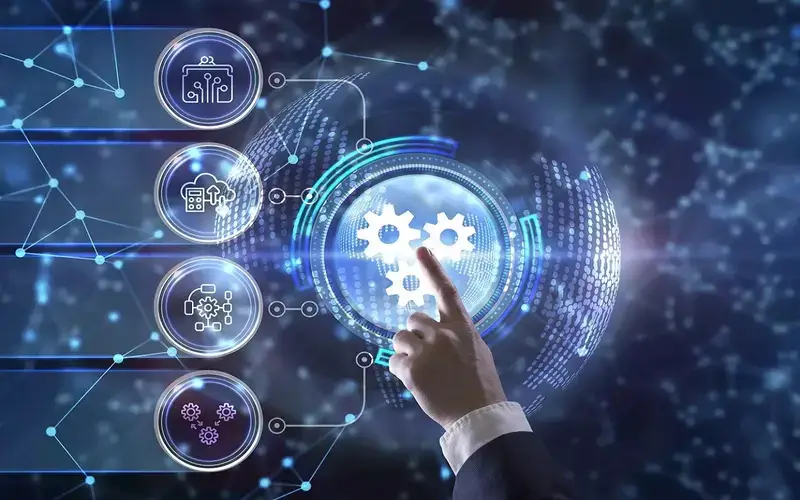
6. Intelligent Perception And Investigation
Sensors are the sensory organs of autonomous systems, crucial to their mobility and autonomy. Data from numerous and even distinct sensors are combined to communicate information quickly and correctly about the environment, physical events, activities, or conditions to decision-making components.
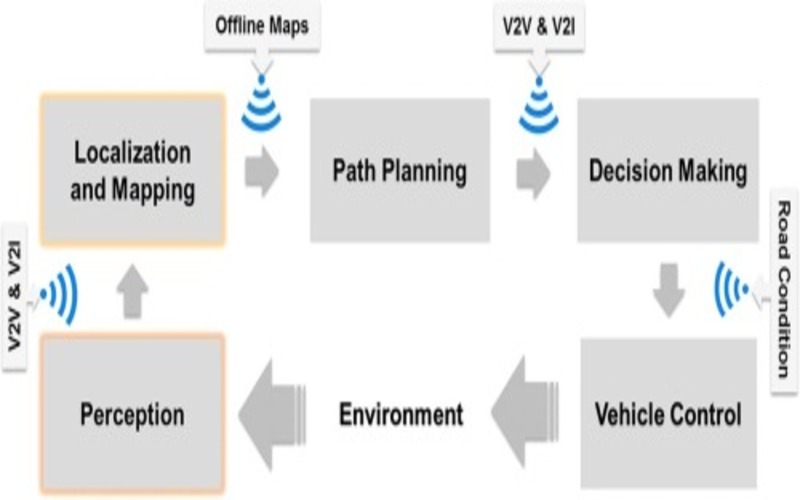
7. Planning, Control And Decision Making
Designing with intelligence is a requirement for autonomous and predictive action. Systems must be able to plan their actions to attain a certain goal and respond to unexpected or new conditions by modifying their behaviour. Machine learning strategies merge sensory data with past background and conclusions that can be used to enhance actions.
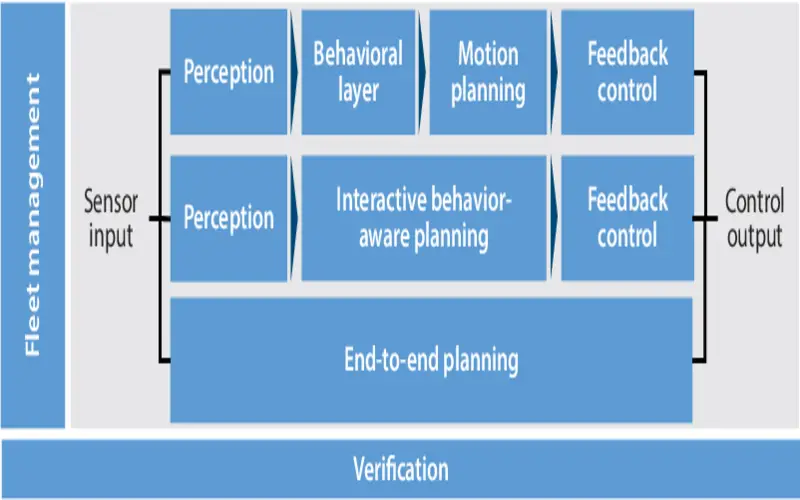
8. Software Strategies And System Development
The development of autonomous systems is reached from a software engineering outlook. Several characteristics of autonomous systems, architectures, models, and languages will be closed and demonstrate the technical credibility of systems that can forcefully adapt their conduct to changes in operating situations using software. The mandatory part is concluded by elective modules. These modules focus on the progress of autonomous systems, automotive applications, technology management, or engineering procedures applied in tech businesses.
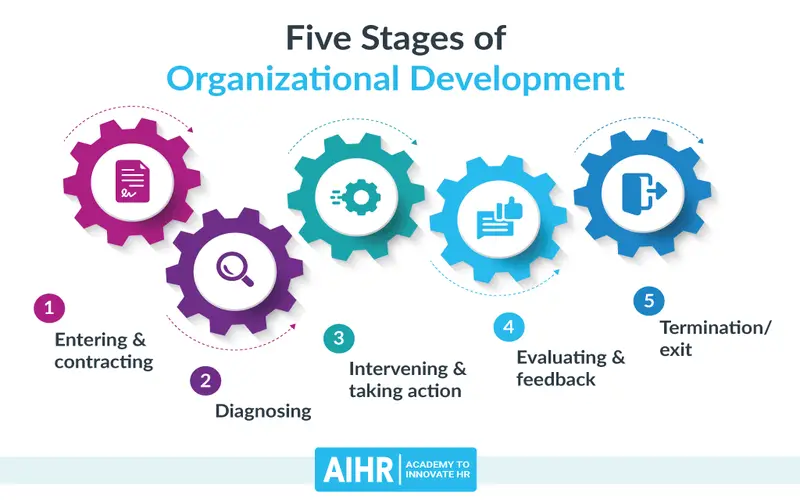
9. Sensor Technology
Enhancements in sensor innovation involving LiDAR, radar, and cameras have improved the capabilities of autonomous systems to recognize and interpret their environments. These sensors are critical for offering actual-time data to the system.
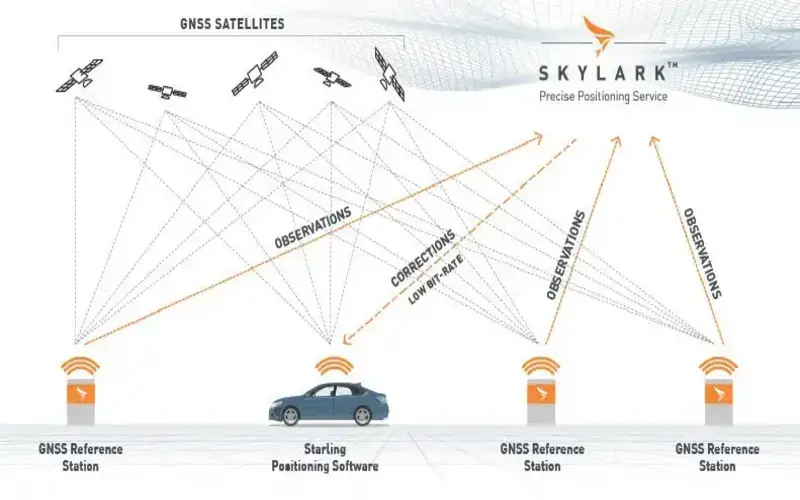
10. Observing, Control, And Task Allocation
A human employee may be on board for testing and risk organization to take over the energetic driving tasks in Avs. The guidelines in the TR do not account for any human-system interchanged and assume a stage 5 automated driving system for the AV. For cooperation industrial when robots work with humans, robots need an observed stop button and organization of a constant speed and distance from human employment.
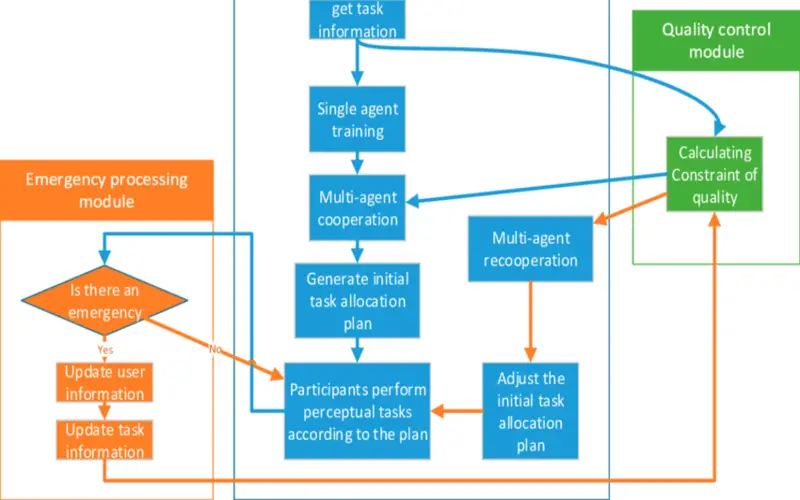
11. Data Organization In The Operation Of Autonomous Systems
The traditional need of each autonomous system with V2x (vehicle to everything) communication policies, encrypted security principles, maps, and provisions for storing, tracking, and updating information on a system. In the mission of Avs appoints data types and formats for the following types:
- Data recording for automated driving.
- The actual use of AV data to enhance safety.
- Organizations of vigorous content like information on road traffic.
- Data for use in examination in case of accidents.
A structured profile for encoding messages defining the compact part of the information, data framing, and messages that outlined header data frame, data molecules, and multiple data frames.
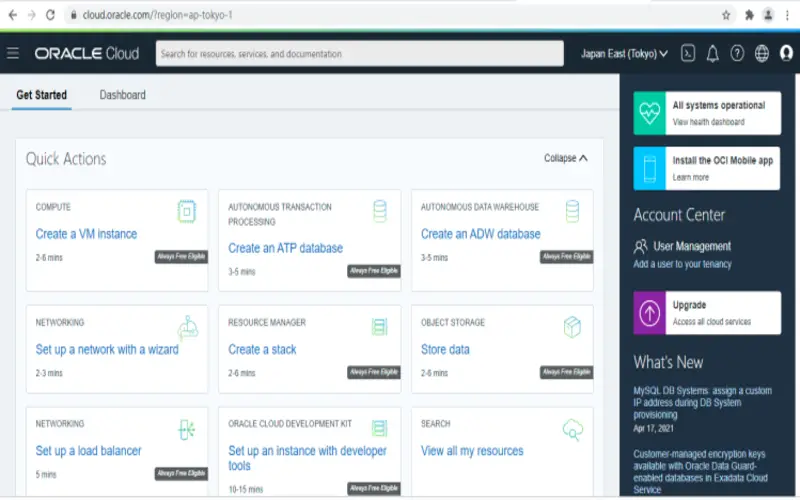