What Is DataOps?
Data operations, or DataOps for compressed, is pre-owned to explain a set of practices and procedures that are structured to enhance the cooperation, integration, and automation of data management operations and duties. These practices and procedures involve a concentration on agile methods. It is planned to help enterprises better organize their data pipelines, minimize the workload and time need to evolve and employ latest data-driven applications and enhance the quality of the data being used.
DataOps is termed to reduce obstacles between data engineers, data experts and data/business evaluates and other group and departments within an enterprise allowing them to work together more effectively to organize and evaluates data.
Why Is DataOps Essential?
In today’s energetic advance companies’ world, DataOps plays an essential role in helping enterprises and organizations stay ahead, as the capability to evaluates data quickly and correctly can give then a competitive advantage over others. DataOps clarifies and automate the complicated procedures of collecting, storing, and monitoring data, making it more effective, accurate and related to the company needs/requirements. It allows businesses to better use their data assets and derive value from them.
Benefits Of DataOps
The benefits of DataOps are several and can have an essential effect on an organization’s data management practices. Some of the critical advantages of DataOps involve:
Enhanced Data Quality: By automating data management processes, enterprises can essentially minimize the risk of human issues and ensure that data is transformed in a timely and correct manner.
Faster Time-to-Insights: DataOps can management minimize the time it takes to withdraw insights from data by enhancing the speed and effectiveness of data transformation.
Greater Agility: By applying agile methods to data management, enterprises can more simply adapt to converts in business needs and react rapidly to merging opportunities.
Maximized Collaboration: DataOps highlight cooperation between data experts, coders, and operations groups, which can assist in breaking down silos and enhance communication.
Better Resource Utilization: By automating data management procedures, enterprises can minimize the amount of time and resources needed to organize and deliver data, which can result in cost savings.
1. Data Governance
The procedures of establishing rules, and traditional for organizing data assets, and an organizational infrastructure to support enterprise data management, in refers as data governance. Data governance in DataOps assists to ensure that data is collected, stored, and used in a consistent and in ethical manner.
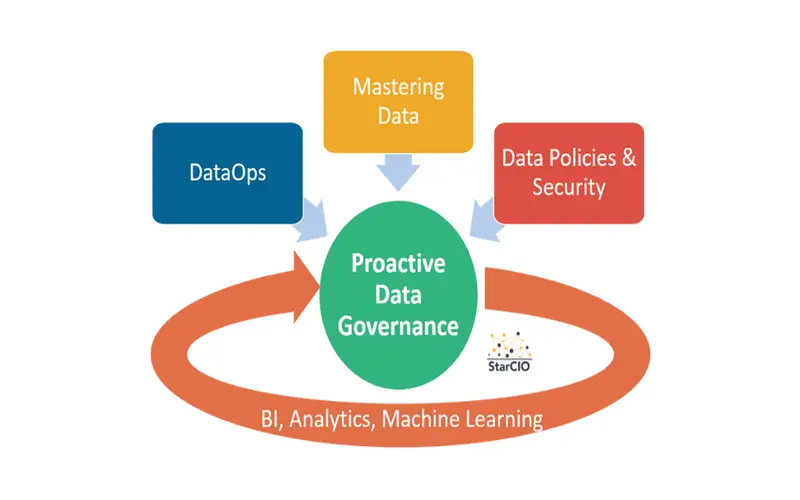
2. Data Quality Management And Measurement
Data quality organization and measurement include recognizing, correcting, and avoiding any issues or paradoxes in data. It ensures that the data being pre-owned is scalable and correct. It is vital because poor data quality can lead to incorrect, inaccurate understanding and decisions, which can have serious difficulties.
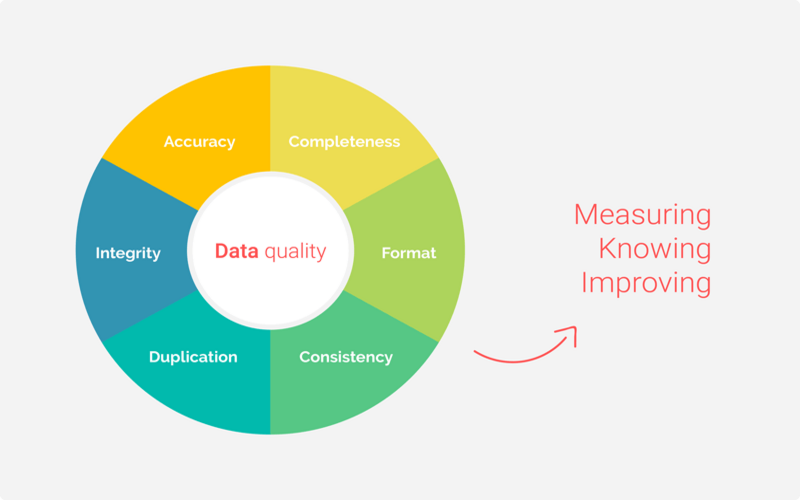
3. Use Table In/table(s) Out Protocols.
DataOps uses table(s) In/table(s) Out protocols, popularized as Data Contracts by Andrew Jones, as a path to clarify data merging by offering a well described interface for data merging.
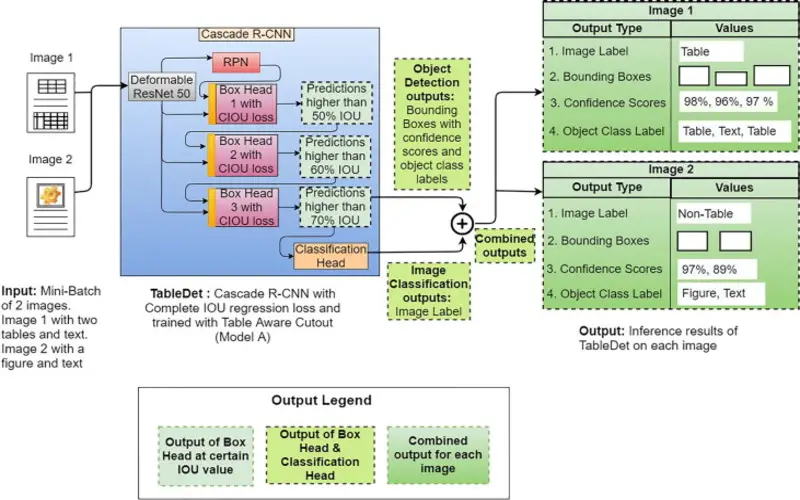
4. Features Deterministic, Probabilistic, And Humanistic Data Integration
DataOps assists various types of data merging, which enables management to select the best approach for a particular data management task. Destined merging is when data is matched based on a set of possible rules, uncertain integration is when data is matched based on credibility, and progressive integration is when a human specialist matches data. It enables faster integration and a transparent separation of commitments between distinct data organization duties.
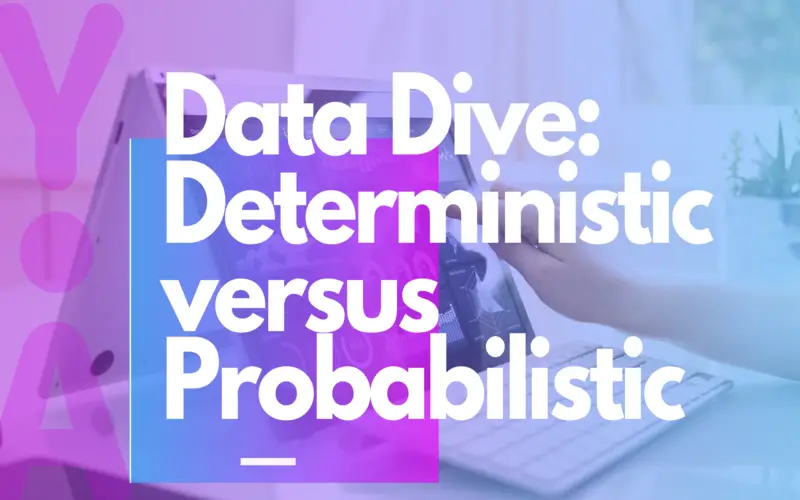
5. Maximized Volume Of Data
Maximized data means more chances and insights but only if that data is governed and controlled appropriately. It groups make finite governance and context around data; it can take weeks or months to surface understanding. Aside from being obsolete by that time, those understanding might not even be correct. DataOps goals to solve the problems of both speed and quality, even as the volume of data most managements has continues to maximizes.
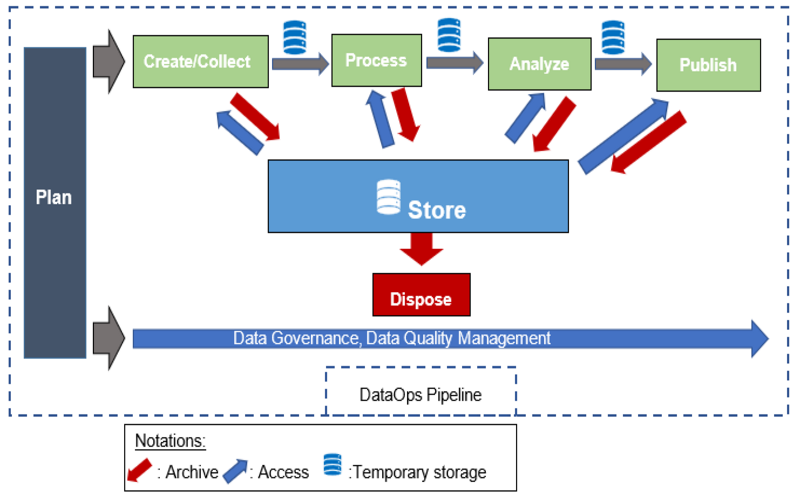
6. Maximizing Systems And Procedures That Depend On Data
In a world where nearly everything depends on data, enterprises cannot afford to have sudden or inaccurate decisions that affect end consumers, company growth, and income. Once again, assists make sure scalability by concentrating on timeliness and accessibility.
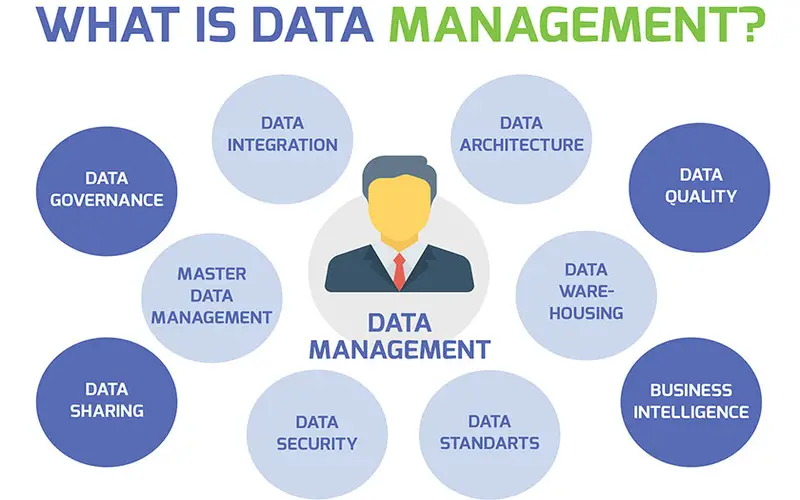
7. Assembling A Skilled team
They are achieved in DataOps articulation no having the right group in place. You will require a different team of data engineers, experts, and evaluates who can work in unity. They should possess skills in data merging, quality control, and evaluates, ensuring an extensive reached to organizing and influencing data.
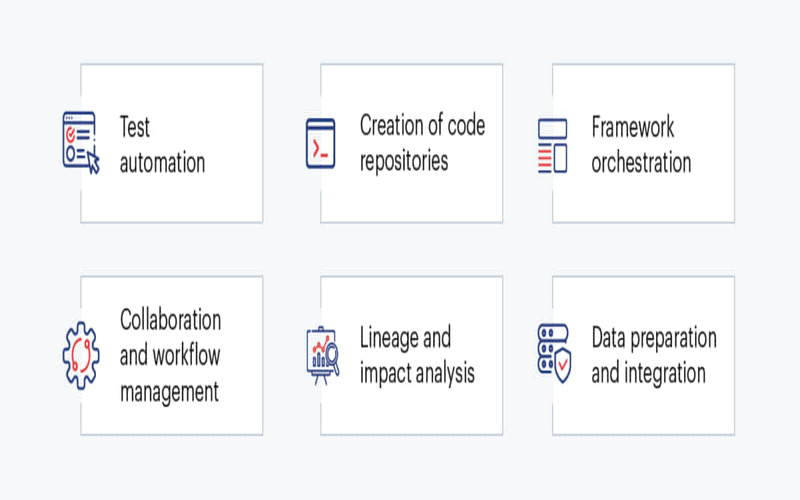
8. Selecting The Right Devices
The efficiency of your DataOps action rely on the depends you use— dataOps for software solutions that benefitted data integration, quality control, and actual-time analytics. The proper innovation stack is not just a developer it’s vital part that can essentially affect the success of your DataOps works. Select devices that improve effectively and fit well with your group’s workflow and your overall company objectives.
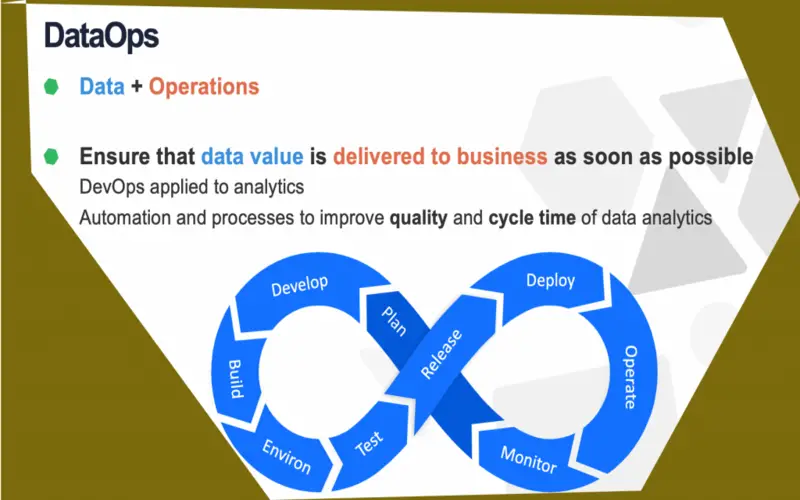
9. Iterative And Incremental Approach
In the data universe, affirmation is a constant difficulty. It’s not just about collecting data, but also ensuring that it is scalable, usable, and aligned with business objectives. Enter repetitive and maximized approach, a cornerstone of Agile methods. In this example, data validation is not a flexible task done once but is broken down into smaller, manageable portion. Each cycle provides an opportunity for recast and fine-tuning, leading to more scalable and tough data ecosystem over time. The practical result of this approach is multifold. Initially, it enables data experts to make rapidly converts in design with the developing companies needs or regulatory landscape.
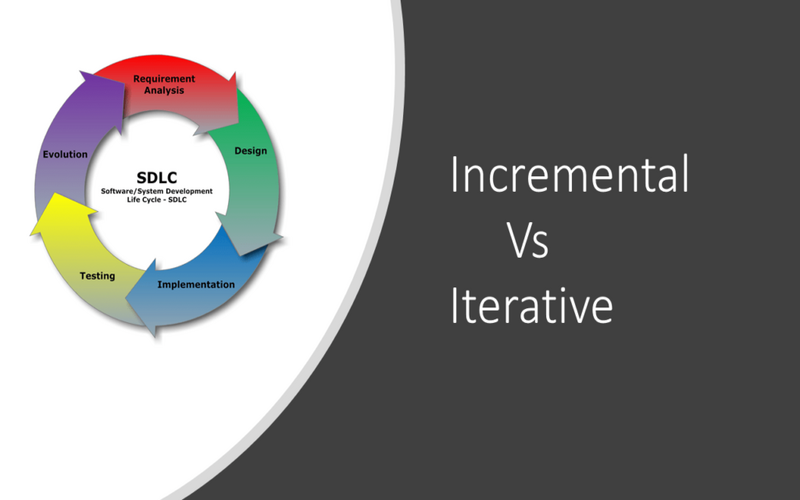
10. Embracing Change
When Agile promote envelop convert, it’s not just a theoretical nicety but a practical essentially, especially in the ever-developing globe of data. Unlike traditional systems, which can be fragile in the face of change, Agile-allowed data structures are designed to adapt. As your management revolve or scales, or as latest data types come into play, the structure steadily a modify. It’s a resilience that’s singly essential is today’s actual-time evaluates driven business world.
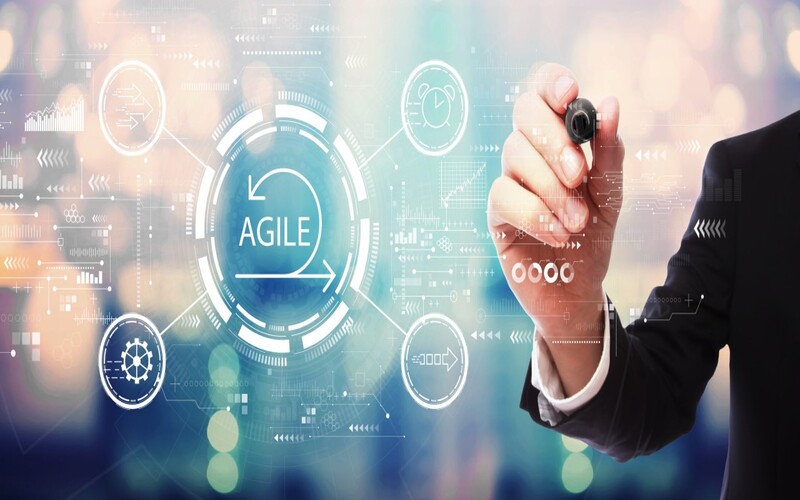