The improvement and unfolding of the Internet and clever gadgets have contributed considerably to a boom in the number of visitors to the web, apps, and SNS systems. In addition, those systems are growing their series of diverse statistics records, which could reveal customers’ preferences. In particular, the lively use of customers’ SNS systems makes it feasible to accumulate an extensive variety of records, which include statistics about fans associated with the consumer, tweet records, and statistics uploaded by the consumer. In addition, the improvement of wearable sensors related to clever gadgets helps the gathering of diverse consumer-associated exercise and bio-associated clinical records.
1. What is a Sequential Recommendation System?
Sequential advice structures attempt to recognize the consumer’s entry over the years and version in sequential order. The consumer enter interplay is largely sequence-dependent. That approach if someone books a flight, it books a taxi additionally for the destination, and books a room. These statistics are saved in sequence. If some other man or woman books a flight and taxi, the gadget will provide tips for lodge or room booking. Both customers’ desire and object reputation are dynamic or it adjustments over the years.
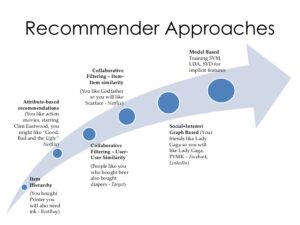
2. How are Sequential advice structures exceptional from others?
In conventional advice structures like Collaborative filtering and Content-primarily based filtering fashions, gadgets interactions are statically and simplest seize the consumer’s popular preferences. But within side the Sequential advice gadget, the object interplay is a dynamic sequence.
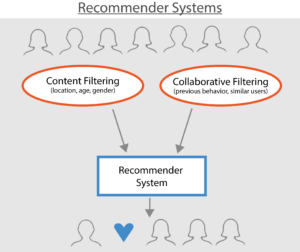
3. When to apply Sequential Recommendation Systems?
In the virtual international, wherein the entirety goes virtual, the consumer’s sequential conduct is a wealth of statistics. A sequential advice gadget may be used within side the area of e-trade to decide the ancient conduct of the man or woman and are expecting non-stop extra de in desire.
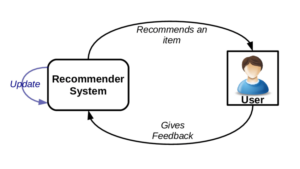
4. How Does it Work?
Generally, a sequential advice gadget takes a chain of statistics from customers and attempts to be expecting the following consumer-object interactions which could appear within side the close to future. Given a chain of consumer-object enter interactions; the version will rank the pinnacle candidate gadgets. This object is generated by maximizing an application feature price.
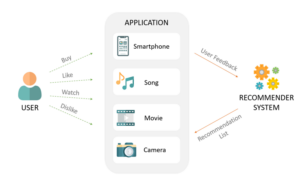
5. Literature Surveys
Recommendation structures are a beneficial generation that could alleviate the hassle of overload of statistics supplied to customers. It predicts the grade of gadgets to be advocated by the consumer, creates a listing of advice ratings for every consumer, and makes it feasible to advocate gadgets associated with the consumer. Several platform offerings actively advocate personalized gadgets that meet the wishes of customers through introducing an advice gadget. To enhance the overall performance of those tips, research on diverse advice filtering fashions and records mining strategies are being conducted.
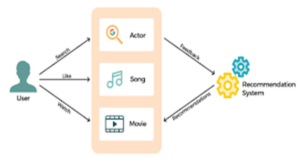
6. Content-Based Filtering
In 1992, beginning with the take a look at Loeb et al, diverse fashions for filtering statistics emerged. Content-Based Filtering is a way for recommending gadgets with attributes just like people who customers like and recommends them primarily based totally on the statistics of the gadgets. That is, it’s far away from recommending comparable gadgets primarily based totally on statistics on gadgets decided on through the consumer within side the past. The Content-Based Filtering version is the maximum primary version in the general advice gadget version and turned into specifically utilized in early advice.
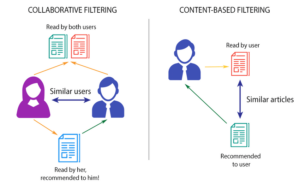
7. Collaborative Filtering
Collaborative Filtering is a statistics filtering version that was first regarded within side the 1990s and has become a stepping stone for the following studies on recommender structures. Collaborative Filtering is a version that constructs a consumer’s desired database the use of the consumer’s assessment records too are expecting gadgets that suit the consumer’s taste, after which makes use of it for advice. This version may be labeled as Memory-Based Collaborative Filtering and Model-Based Collaborative Filtering. Memory-Based Collaborative Filtering may be similarly divided into User-Based Collaborative Filtering and Item-Based.
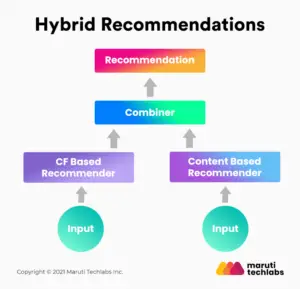
8. Data Characteristics And Challenges
As the conduct of clients, for example, purchasing is numerous and complicated in a real-international scenario, exceptional records enter traits from clients will carry exceptional demanding situations. Some of them are indexed within side the beneath the table.
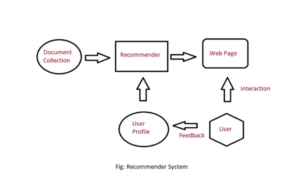
9. Sequential Recommendation System
Sequential advice structures upload greater prices to many industries and the gadget itself may be very consumer-pleasant because it recommends what subsequent motion has to be taken. It is used within side the e-trade enterprise to advocate the following applicable product to clients.
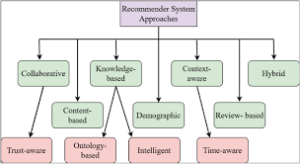
10. Final Words
In this article, we’ve got were given primary know-how of sequential advice structures and will recognize the way it works. We additionally had clean know-how of the distinction among conventional and sequential advice structures. In the end, we additionally mentioned the demanding situations confronted through the sequential advice gadget in conjunction with its applications.
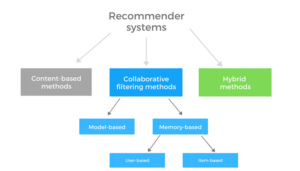