For the past few years, top tech companies have been chasing AI and machine learning. They have been working towards developing processors for AI and deep learning. Every industry wants to incorporate AI into their business. An AI chip, also known as an AI accelerator, is a specialized integrated circuit designed to efficiently process machine learning tasks using programming frameworks like Tensor Flow and PyTorch. These chips are useful in applications inspired by the human brain. Usually, an AI chip consists of Field-Programmable Gate Arrays, Graphic Processing Units, and Application-Specific Integrated Circuits. They perform complex tasks the way we human beings would complete. They accelerate calculations and task computation required by AI algorithms. Designing AI applications requires chips with specific architecture to support deep learning. These chips can be used in smart devices, telecommunication sectors, and more. Within the next five years, we can expect a massive growth in the supply of AI chips. So, without extending long, Let’s get deep down to the Top 10 AI chips already ruling the market.
1. IBM Telum Chip
IBM’s Telum chip tackles fraudulent activities with the help of ML techniques. Its low latency capability enables easy detection of fraud in real time. The process of identifying and computing the hustle is sometimes time-consuming. It can be solved using the innovative features of the AI chip. It has a total of eight processor cores that run at an impressive speed of 5.5GHz. It has a total cache memory of 256 MB.
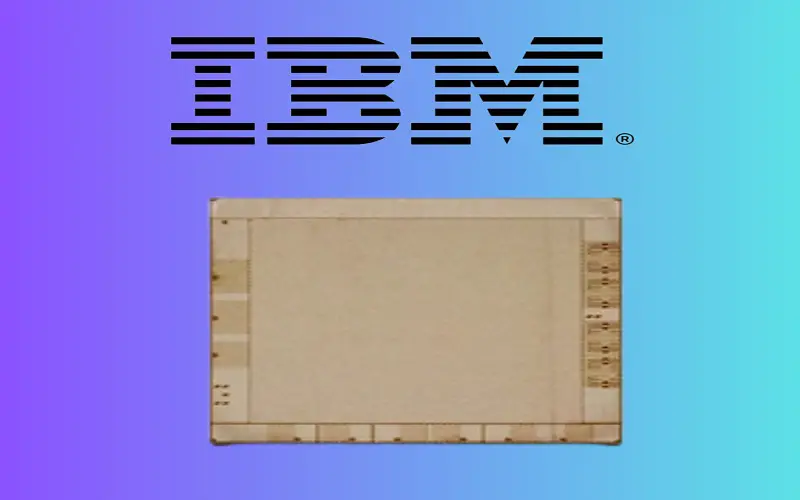
2. Cerebras WSE-2
Cerebras Wafer-Scale Engine-2 is programmed independently for tensor-based linear operations and neural network training. It is the largest AI chip manufactured, being 56X more considerable than the size of the most extensive CPU. It offers 123 times more computing cores and a thousand times more performance on memory chips. It is made with a 7nm fabrication process using 2.6 trillion transistors and 40 GB on-wafer memory.
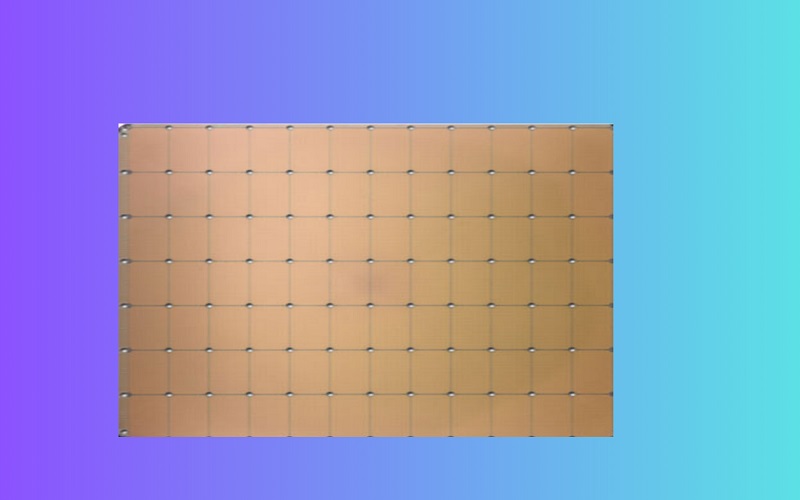
3. Envise
Envise combines photonics and transistor-based systems in a single computation model. It utilizes a silicone photonics processing core for the majority of computational tasks. It is built on 16 Envise chips using 3kW power for server configuration. Each envise chip has 500MB of SRAM for executing the neural networks. With 256 RISC cores per processor, it is ideal for autonomous vehicles, cancer detection, and vision control.
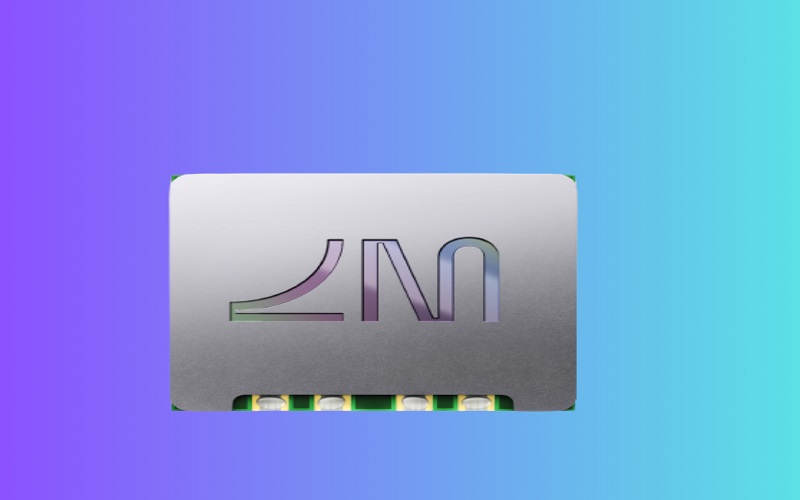
4. Grayskull
With an impressive 120 Tenstorrent proprietary Tensix cores, Grayskull has the fastest interface for handling workloads from edge devices to data centers. Each core has a high-capacity packet processor, a powerful math computational block, a single instruction programmable multiple data processor, and five robust compute cores. It supports 16GB of DRAM, 120MB of SRAM, and 16 lanes of PCle Gen4.
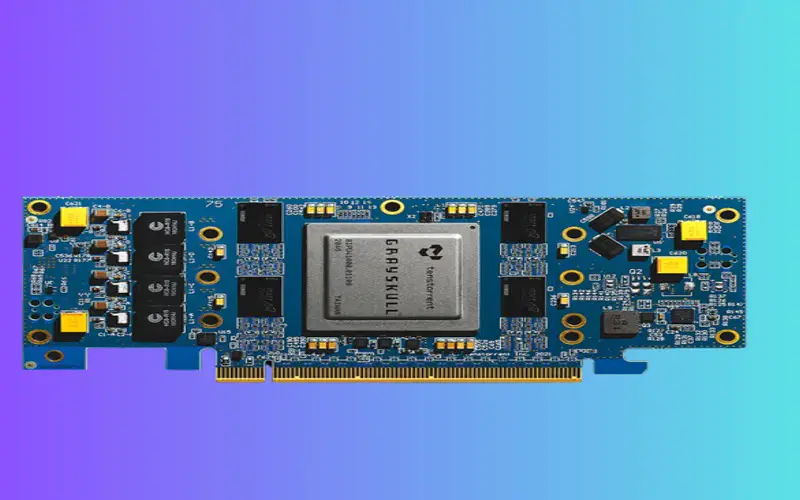
5. Groq Chip
Groq Chip is used to enhance the computing efficiency for ML and AI applications. One of the unique features of Groq is its simple architecture, in which all functions are merged into a single compiler. A compiler is a small chip part that offers space for memory and AU operations. It utilizes machine learning for tracing patterns and optimizing cache for future use. It primarily supports automotive and data centre applications.
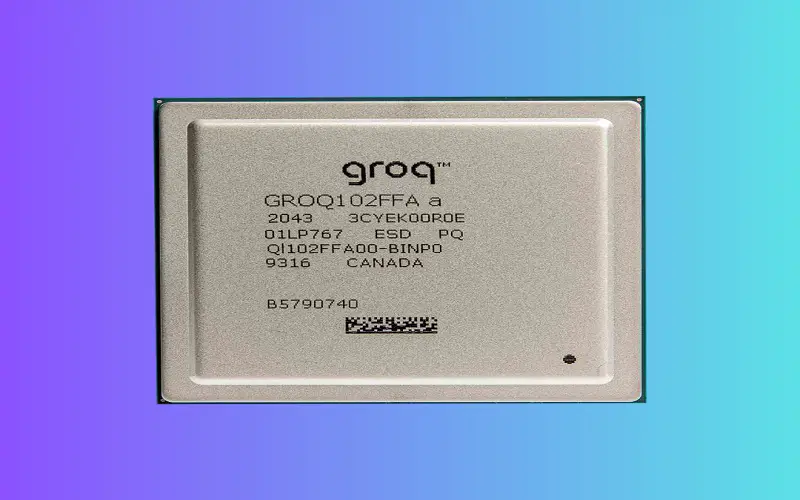
6. NVIDIA A100 SXM GPU 40GB GPU Boards
The NVIDIA A100 SXM GPU 40GB GPU Board is the most potent AI chip with a memory of 80GB. The GPU can handle the most complex calculations with maximum speed and accuracy. It also tackles the latency issue by working on large data sets efficiently. NVIDIA chips are primarily used in labs and data centres.
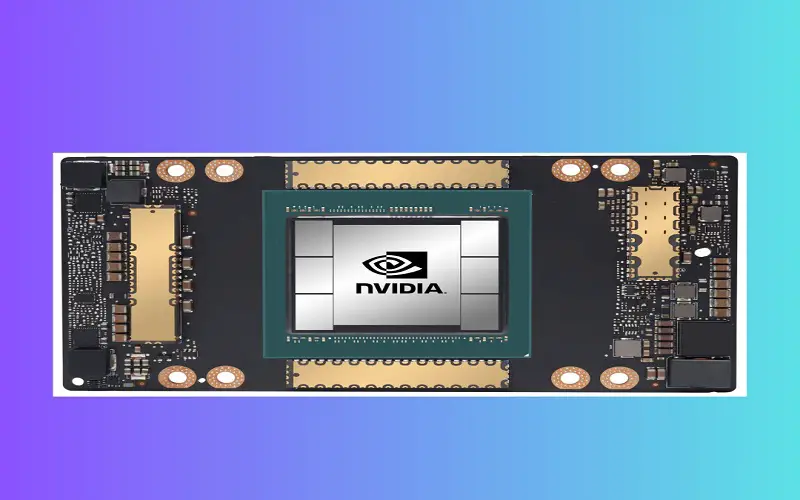
7. Mythic MP10304 Quad-AMP PCIe Card
Mythic’s PCle Card is developed with four M1076 Mythic analog matrix processors capable of delivering 100 TOPSF AI performance while handling workload at a mere 25W of power. It deploys smaller DNN models for processing images from multiple cameras. It boasts an on-chip storage with 4-lane PCIe 3.0 support and a bandwidth of 3.9GB/s. Mythic chips are mainly used in network video recordings and edge appliances.
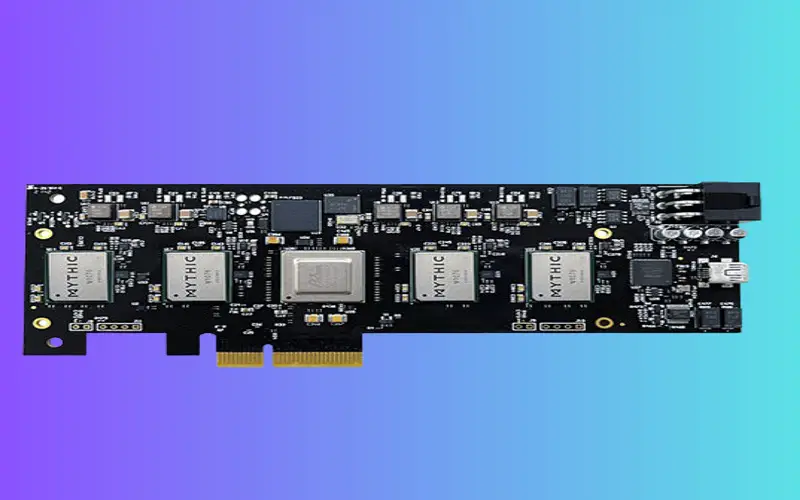
8. Google Tensor
Google has created innovations in the world of smartphone technology by introducing Google Tensor chips for their Pixel devices. All the smartphone functions are combined into this single chip. It has also incorporated machine learning processes for improving visual and speech recognition. With Google Tensor, users can expect high-quality photos and videos and more accurate speech recognition. Tensor has introduced AI and ML to bring cloud computing into the smartphones.
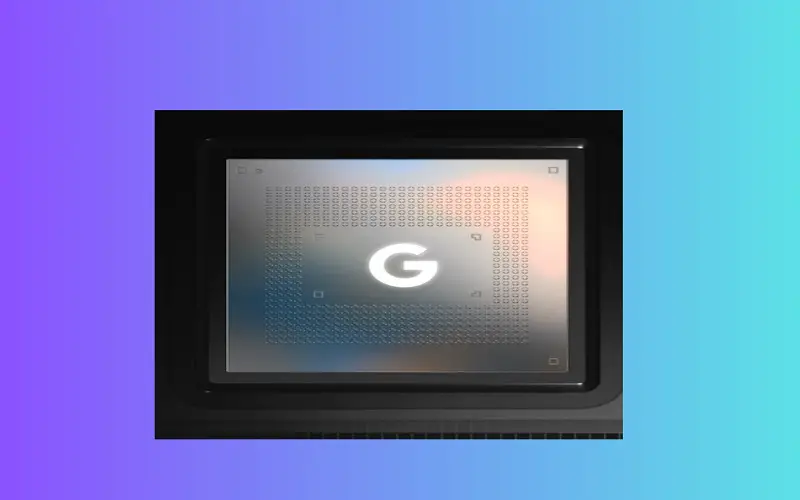
9. 11th Gen Intel® Core™ S-Series
The 11th Gen Intel® Core™ S-Series offers best-in-class wireless and wired connectivity for improved performance. Its core processors are built on the Intel vPro® platform for advanced remote management and enhanced hardware security for the IT industry. By utilizing Intel® Deep Learning Boost, it helps in improving overall AI performance. It also has Gaussian Neural Accelerator 2.0 to suppress the background noise and blurred background videos.
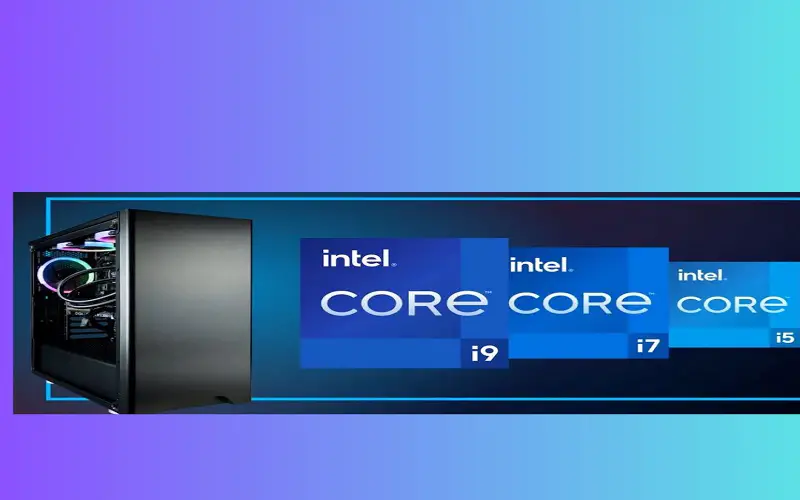
10. Cloud AI 100
Cloud AI 100 has been developed to meet cloud computing requirements, such as the advancement of process nodes, power optimization, signal processing, and more. It is created with a 7nm process and has 16 Qualcomm AI cores to achieve up to 400 TOPs. It has four memory controllers, each running four 16-bit channels with a total bandwidth of 134GB/s. It also supports industry standards using a comprehensive set of SDKs. It enables easy edge-to-end inference in data centers.
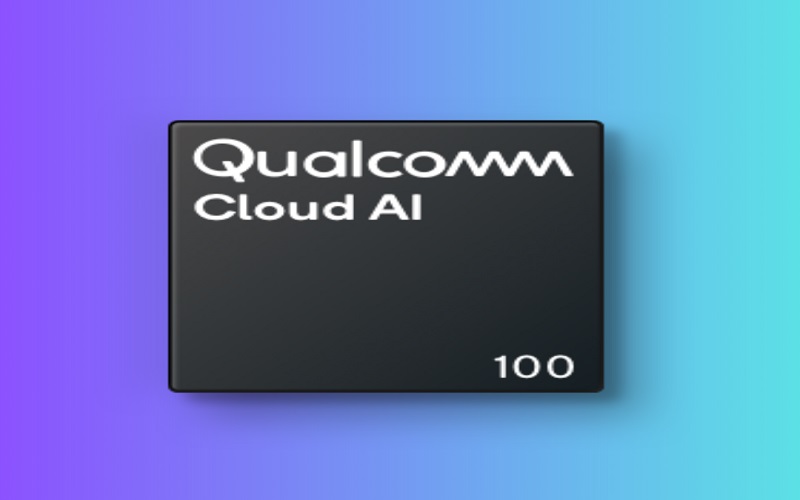