A field of study known as “neuromorphic engineering” aims to develop artificial neural networks that are modeled after the structure and functionality of the human brain. The domains of robotics, computers, and healthcare are just a few that these technologies have the potential to revolutionize. “Neuromorphic computing” is a method of computer engineering that models a computer’s internal workings after the human nervous and nervous system. The expression pertains to the process of developing both software and hardware parts for computers. There are instances where neural computing is referred to as neuromorphic engineering. The top ten advances in neuromorphic engineering are examined in this article, with a focus on breakthroughs that have advanced cognitive computing and artificial intelligence.
1. Spiking Neural Networks (SNNs)
Spiking neural networks (SNNs) imitate the discrete electrical pulses or spikes that actual neurons use to transmit information during communication. Low-power, event-driven computing can now mimic the functions of the brain thanks to SNNs. The development of SNN has improved our understanding of brain-inspired computations and improved the performance of neuromorphic devices.
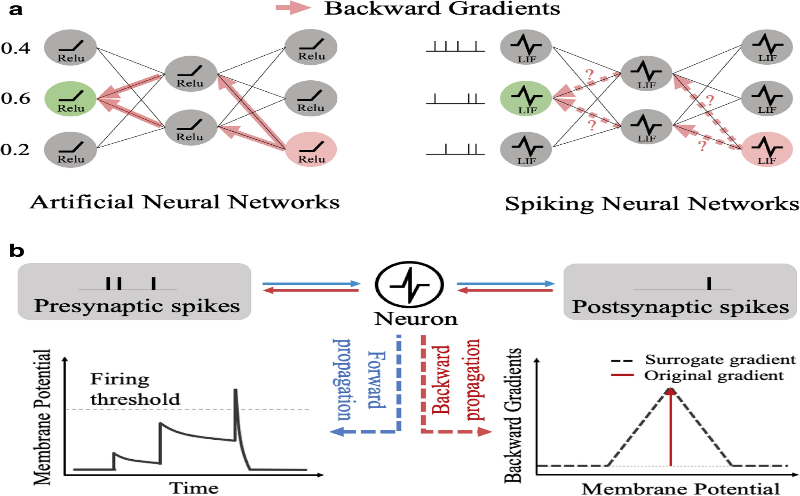
2. Memristors
Electronic parts called memristors imitate the varying resistance of synapses in organic brain networks. These devices can continually process and store data, making them essential parts of scalable and effective neuromorphic technology. Memristor technological advancements have made it possible to create durable and energy-efficient neuromorphic computing devices.
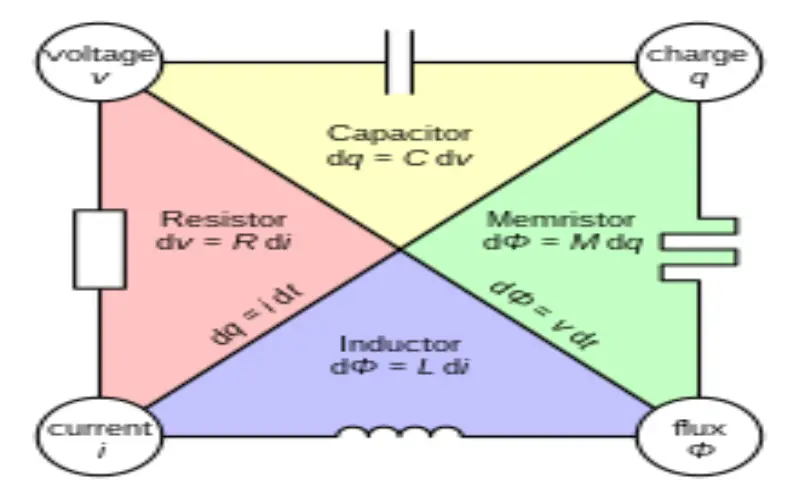
3. Neuromorphic Vision
Machines that use neuromorphic vision systems may sense and process visual data in real time by mimicking the way the human visual system works. These systems process vision with minimal latency and great dynamic range thanks to event-based sensors that only record significant changes in the visual scene. Advancements in augmented reality, surveillance, and driverless cars have all been made possible by developments in neuromorphic vision systems.
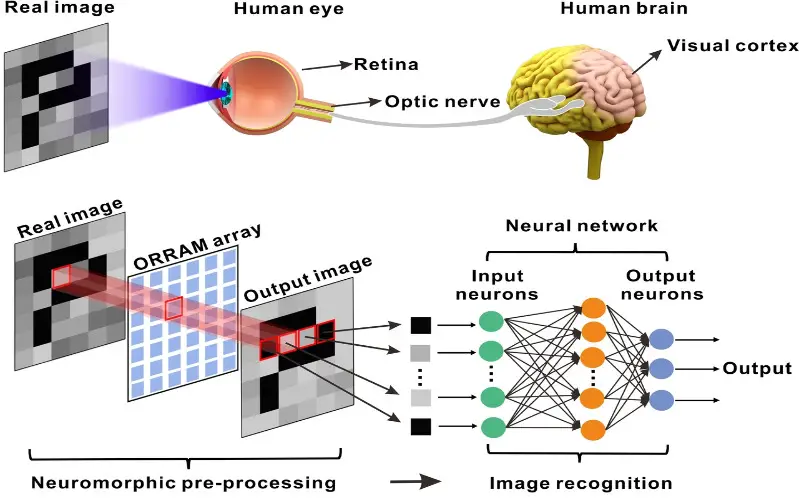
4. Neuroplasticity
The term “neuroplasticity” describes the brain’s capacity to change its physical composition in response to experience. Learning and adaptability are made possible by neuromorphic technologies that mimic this capacity. The development of self-learning and adaptable neuromorphic systems has been aided by advances in the application of neuroplasticity processes, including synaptic plasticity and spike-timing-dependent plasticity, opening up new opportunities for artificial intelligence.
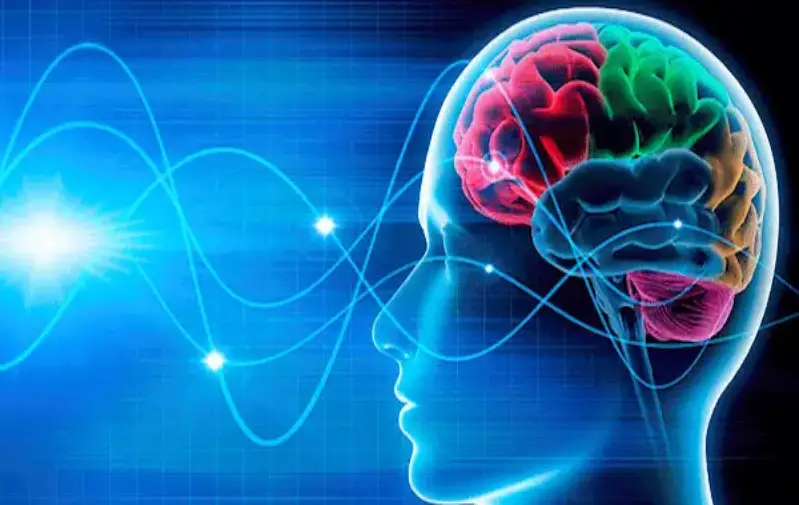
5. Neuromorphic Robotics
Neuromorphic engineering aims to apply insights from neurobiological knowledge to develop next-generation artificial intelligence for computation, sensing, and control of robotic systems. There has been a rapid development of neuromorphic engineering technologies for robotics due to several developments.
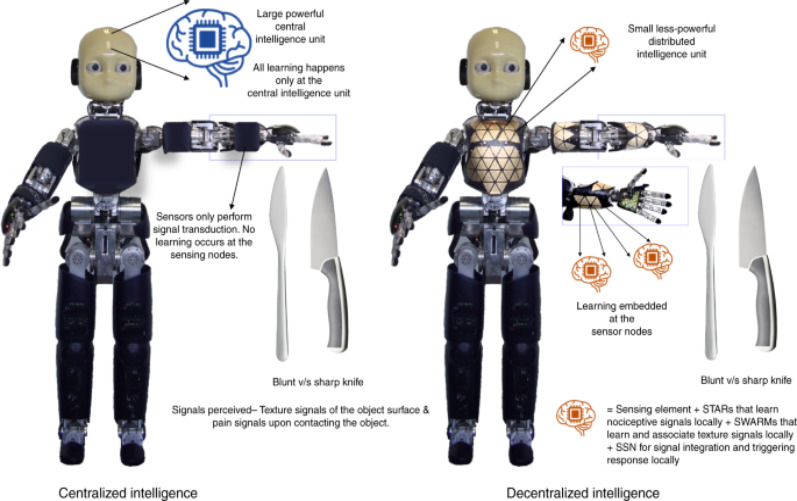
6. Brain-Inspired Computing
Brain-inspired computing entails creating computer models and algorithms that imitate the parallelism and effectiveness of the brain. Novel computer designs, such as neuromorphic devices and processors, have been created as a result of advancements in this field. These architectures can handle complicated calculations more quickly than older von Neumann architectures. Intuitive systems’ development might be accelerated and AI could be revolutionized thanks to brain-inspired computing.
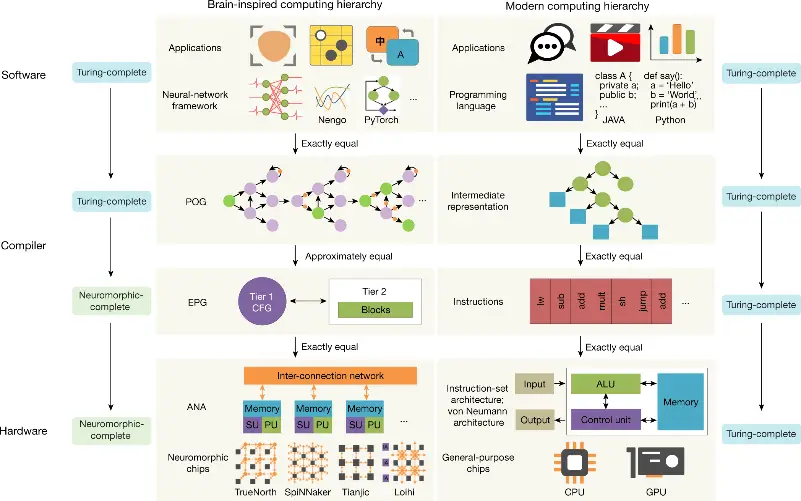
7. Hybrid Neuromorphic Computing Systems
As a result of its distinct temporal, event-driven properties, Spiking Neural Networks (SNNs) are regarded as the third generation of artificial neural networks. In contrast to conventional Machine Learning (ML) techniques, SNNs may exhibit orders of magnitude power efficiency on neuromorphic hardware by utilizing bio-plausible spike-based computing between neurons along with sparse on-demand computation.
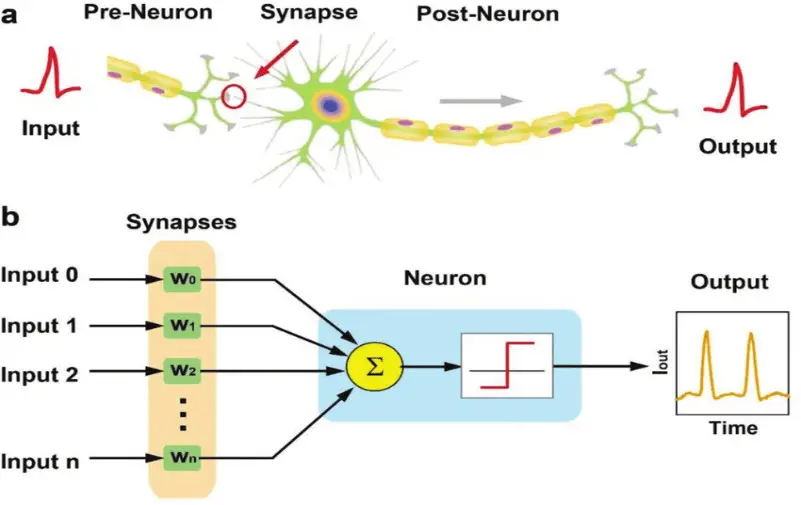
8. Neuromodulation
The term “neuromodulation” describes the brain’s capacity to control neuronal activity and behavior via releasing chemicals. Dynamic control and adaptation of neurological processes are made possible by neuromorphic devices that replicate this neuromodulatory capacity. Artificial neural networks now have greater flexibility and adaptability due to advances in neuromorphic neuromodulation, allowing them to adjust to shifting surroundings and workloads.
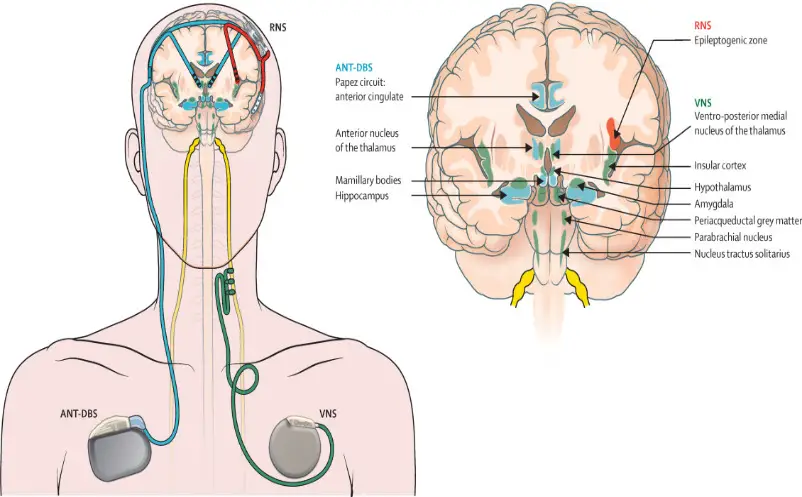
9. Neuromorphic Sensory Integration
Multiple senses, including vision, hearing, and touch, are combined through neuromorphic sensory integration to produce a thorough comprehension of the world. The creation of multimodal neuromorphic systems, which can analyze and integrate data from numerous sensors and allow robots to perceive and interact with the world more like humans, is the result of advancements in this field.
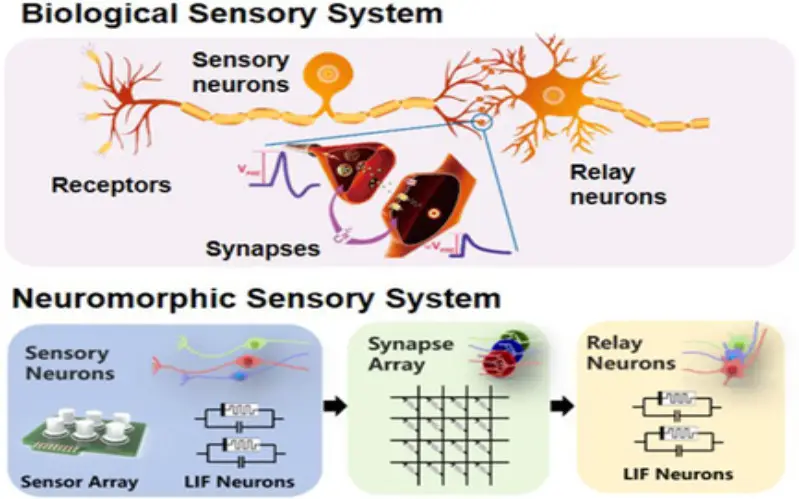
10. Cognitive Architectures
Cognitive architectures develop a model similar to the natural cognitive system of human beings. It focuses on the idea of clubbing cognitive function with AI. It is currently being used widely and much research is still going on to make it useful in the future.
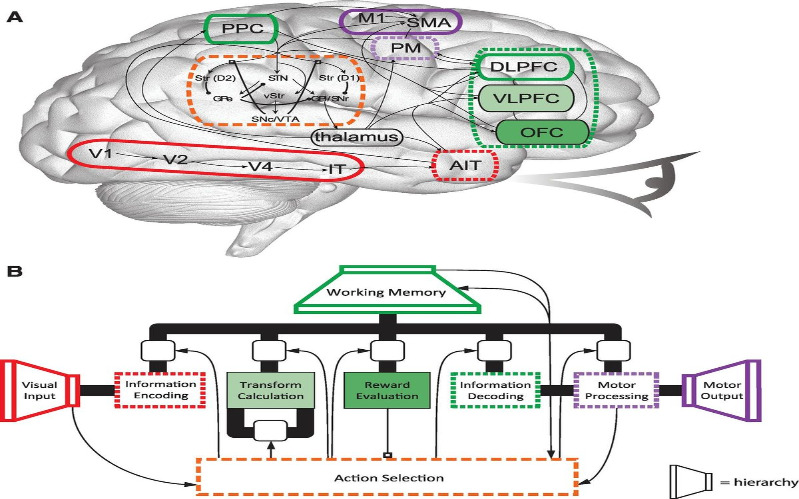