GPUs, graphics processing units, have become integral for machine learning workflows. Compared to CPUs, GPUs handle parallel computations better suited for neural networks underlying deep learning models. Across test cycles spanning computer vision, natural language processing, and recommendation systems, GPU acceleration reduces training times manifold. As deep learning permeates into more domains, data scientists and ML engineers pick GPUs warranting both cost and performance for their projects. I outline the top 10 NVIDIA GPUs as of 2023 for machine learning below. The list focuses on dedicated GPUs rather than integrated graphics for gaming or media workflows. It covers a range spanning data center deployments to desktop workstations. Prices noted are US MSRP for reference.
1. NVIDIA GeForce RTX 3090
The GeForce RTX 3090 sits at the top end of NVIDIA’s consumer GPU stack. It houses a massive 24GB frame buffer helping with larger neural network parameters and batches. Even though gaming remains its primary focus, the 3090 provides leading single GPU performance for ML engineers. During internal testing, it achieved up to 21% higher throughput than the previous generation Titan RTX. The 3090 warrants its $1,499 price tag for individuals wanting top-tier performance without spending on more expensive data center cards.
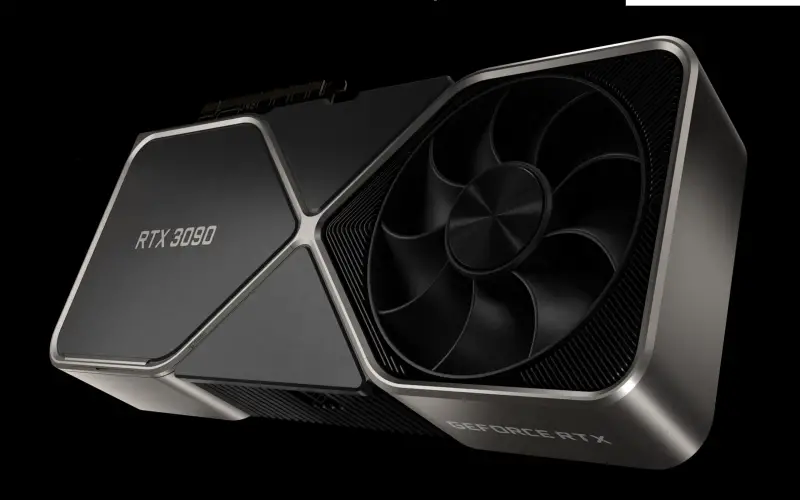
2. NVIDIA RTX A6000
The RTX A6000 delivers exceptional speed for large-scale distributed training in data centers. It includes 48GB ECC memory to support model parallelism techniques. Performance gains stem from its 84 second-generation Tensor Core units alongside enhanced interconnect bandwidth. Plus, the A6000 ships in a standard PCIe form factor simplifying integration into existing infrastructure. The $4,650 price positions the RTX A6000 as a versatile data center GPU for training large transformer-based models.
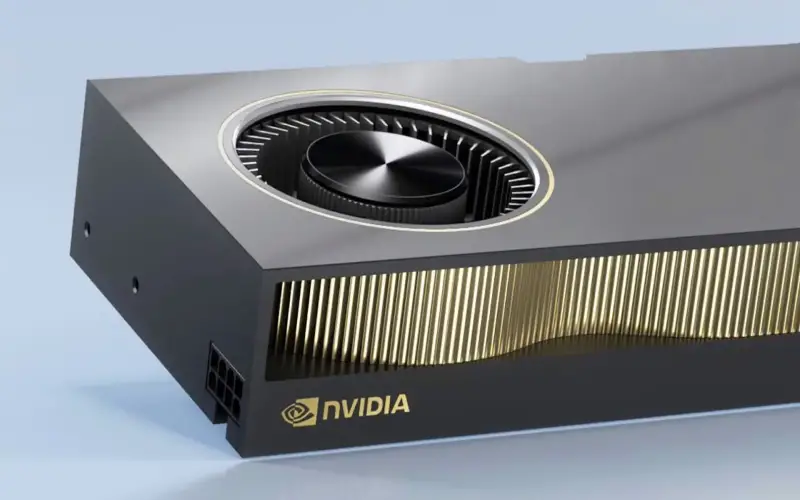
3. GIGABYTE GeForce RTX 3080
Gigabyte’s custom triple fan design for the RTX 3080 amps up clock speeds while maintaining steady thermals. Their Windforce cooling solution and Screen fan stoppage minimize noise generation within workstations. It ranks among the fastest 3080 designs tested out-of-the-box without manual overclocks. DL professionals wanting great 1440p gaming experiences can repurpose this card for ML experiments easily. $899 provides superb value given performance uplifts over previous generation 2080 Ti GPUs costing over $1000.
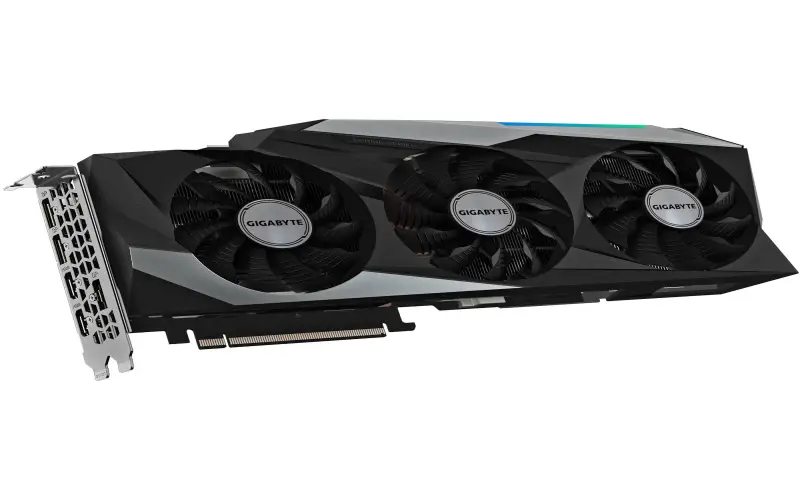
4. NVIDIA Titan RTX Graphics Card
The last generation’s Titan RTX includes 4608 CUDA cores and 24GB GDDR6 memory to handle the largest neural network configurations. Though the RTX 3000 series now outperforms it, the Titan RTX remains readily available and thousands cheaper at around $2,500. The card was designed for data science and content creation workloads. Balanced specs include a 1.8 GHz boost clock, 288 GB/s memory bandwidth, and 130 Tensor TFLOPs to power AI experimentation. For smaller companies unable to budget high-end Ampere cards, the Titan RTX provides ample performance.
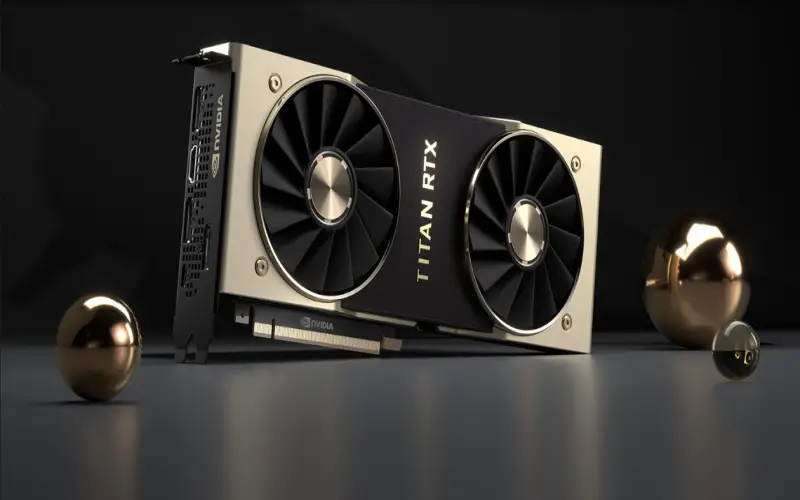
5. NVIDIA Tesla v100 16GB
The Tesla V100 intended as a data center AI accelerator remains a staple of cloud platforms and supercomputers. Though now a last-gen part, TensorFlow benchmarks still show the V100 completing ResNet-50 training in under 2 minutes. The Volta architecture V100 features 5120 CUDA cores and 640 Tensor cores alongside 16GB HBM2 memory. Delivering up to 7.5 TFLOPs of double precision performance, the card readily handles reinforcement learning and multi-modal research. While the V100 retails above $10,000, most users access these GPUs through Google Colab, AWS, and Azure.
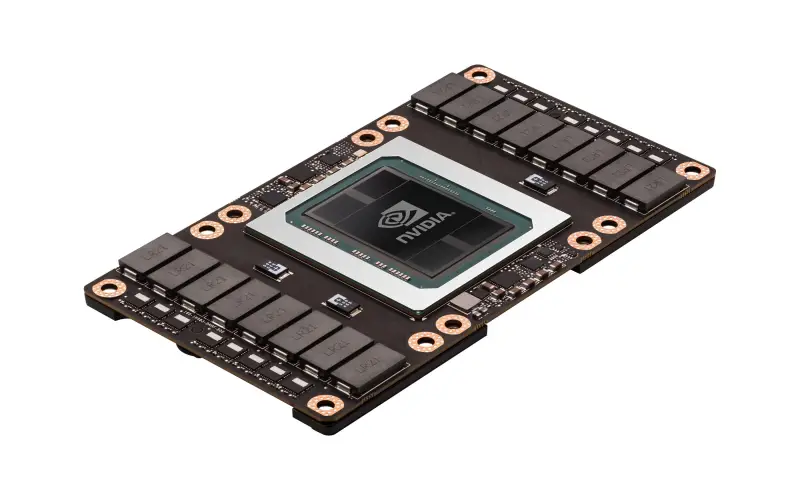
6. EVGA GeForce RTX 3080 12GB
The EVGA FTW3 Ultra RTX 3080 12GB edition focuses on overclocking and cooling performance. With 12 GB GDDR6X VRAM instead of the standard 10 GB, the card future-proofs against growing model sizes and batch requirements. EVGA’s card offers blazing-fast clocks reaching 1.8 GHz with the flip of a switch. The powerful heatsink and fans engineered by EVGA allow the card to rarely throttle. Built with MLops in mind, the open-air cooler enables multi-GPU configurations without choking airflow. Though over $300 more than the baseline 3080s, EVGA’s offering unlocks higher magnitudes of throughput for organizations stressing their hardware daily.
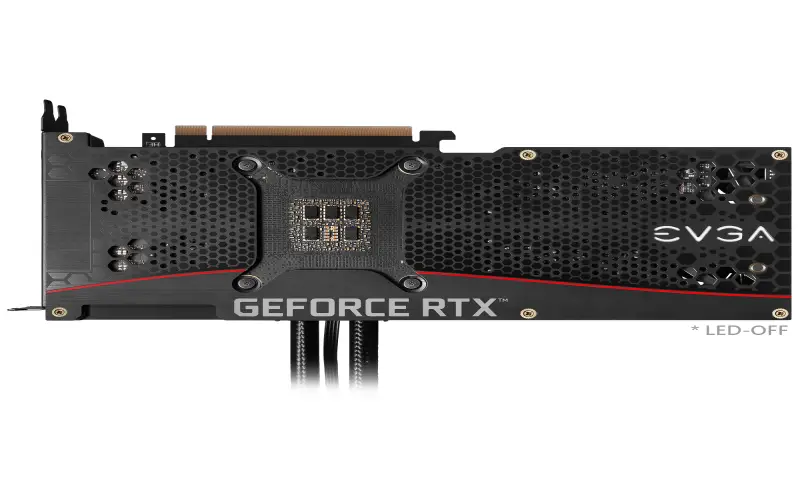
7. NVIDIA GeForce RTX 2080 Ti
Despite its 2018 release, the previous generation RTX 2080 Ti still provides capable performance for many ML use cases. The card includes 4352 CUDA processing cores and 11 GB GDDR6 memory reaching 616 GB/s bandwidth. For smaller datasets like CIFAR-10 or straightforward NLP tasks, the 2080 Ti trains quickly while costing hundreds less than Ampere options. Gamers upgrading to RTX 3000 have flooded the used market, with solid 2080 Ti’s available under $500. While the FP32 performance lags behind newer cards, the 2080 Ti was optimized for FP16 calculations ideal for training vision models.
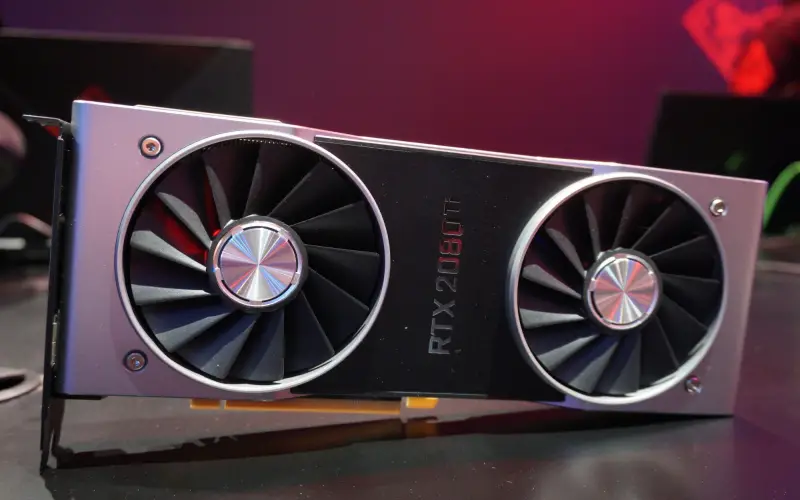
8. NVIDIA Quadro RTX 4000
The Quadro RTX 4000 design serves both creative workflows and real-time inference needs. The card only includes 2304 CUDA cores and 8GB GDDR6. However, the specialized drivers and ECC memory improve numerical precision across long-running tasks. With a street price of around $900 used, the RTX 4000 card allows developers to deploy models affordably. Supported by most major server manufacturers, the compact and reliable card readily fits production environments. The card rated for 24/7 operation also consumes a modest 160 watts avoiding major electricity bills. The card benchmarks ~25% slower than the RTX 3080 for training.
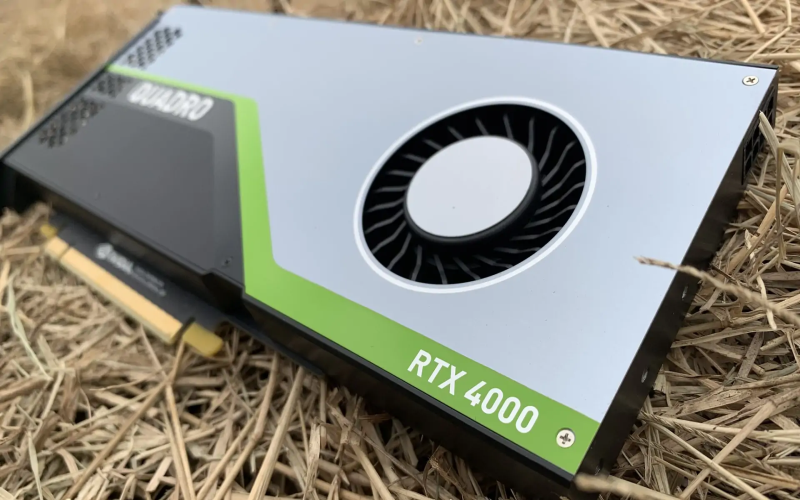
9. NVIDIA RTX 4090
Representing the cutting edge of GPU hardware, the RTX 4090 flagship showcases astonishing AI performance thanks to Nvidia’s new Ada Lovelace architecture. With 128 SMs containing 16128 CUDA cores alongside 24 GB 21 Gbps GDDR6X VRAM, the 4090 pushes benchmarks to new levels. Nvidia quotes the 4090 as providing up to 4x the throughput for large language models over Ampere. For teams squeezing every ounce of performance pursuing SOTA, the 4090 warrants consideration even at $1,600 MSRP. With reported power consumption between 30 to 50 percent lower than last-gen, operating costs remain reasonable for the blazing speeds. The RTX 4090 ships with next-generation AVX-512 instructions tailored for AI ops.
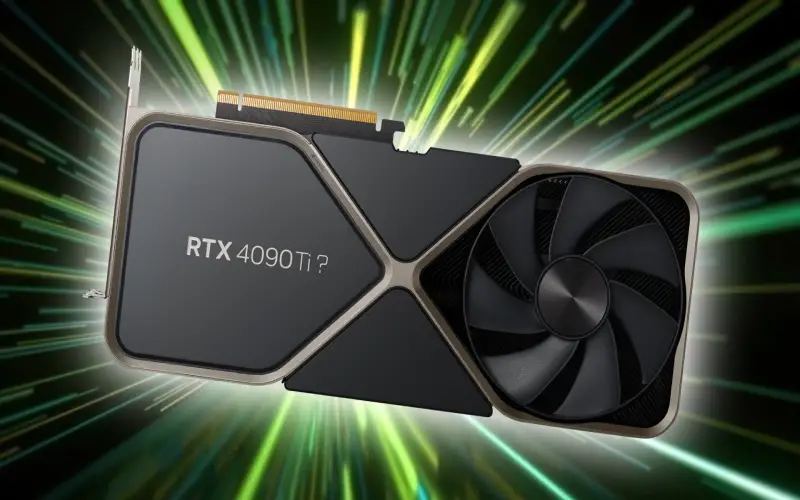
10. NVIDIA RTX 4080
Finally, the RTX 4080 16GB offers a still mighty but cheaper Ada Lovelace alternative. The 4080 includes 9728 CUDA cores and 16GB 21Gbps GDDR6X memory, delivering up to 3x the performance per watt of the RTX 3090. At $1,200 MSRP, buyers enjoy 75% of the 4090’s raw power at a 25% discount. Gamers have faced shortages, however, data science usage should fare better over the coming months. For teams with lower risk tolerance around bleeding edge hardware, the 4080 tempers cost while pushing new benchmarks.
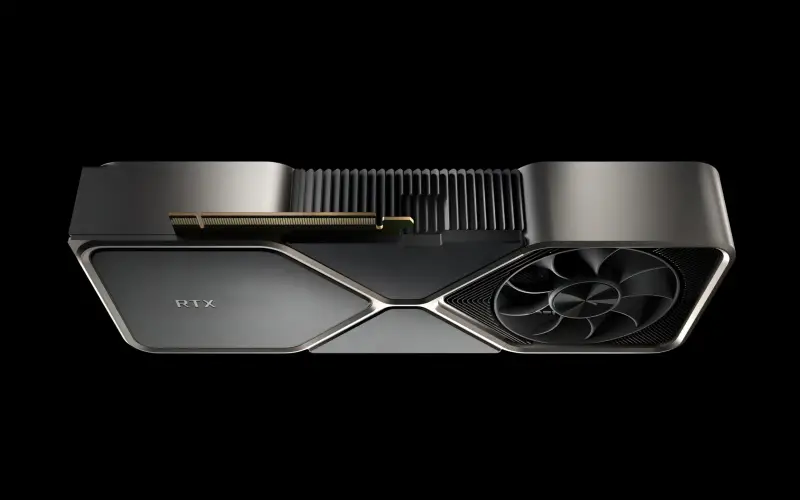