Machine Learning (ML) is already most famous technologies in the present time and is used in most of the fields. But what about those who don’t know well about ML? That’s where Automated Machine Learning or AutoML or Automated ML comes into the picture. It is defined as the procedure of automating the tasks of applying ML to real-world problems. AutoML permits data scientists, machine learning enthusiasts, analysts, researchers, and developers, to build machine learning models with high scale, efficiency, and productivity. And for performing these AutoML tasks, there is a need for the best AutoML software. Therefore, we are here to help you know about the top 10 AutoML software and tools.
1. Auto-Keras
It is an open-source AutoML software based on Keras to make ML (machine learning) accessible to everybody without prior knowledge of ML models and applications. It was developed by DATA Lab at Texas A&M University and community contributors. Auto-Keras offer functions to auto search for architecture & hyperparameters of deep learning models.
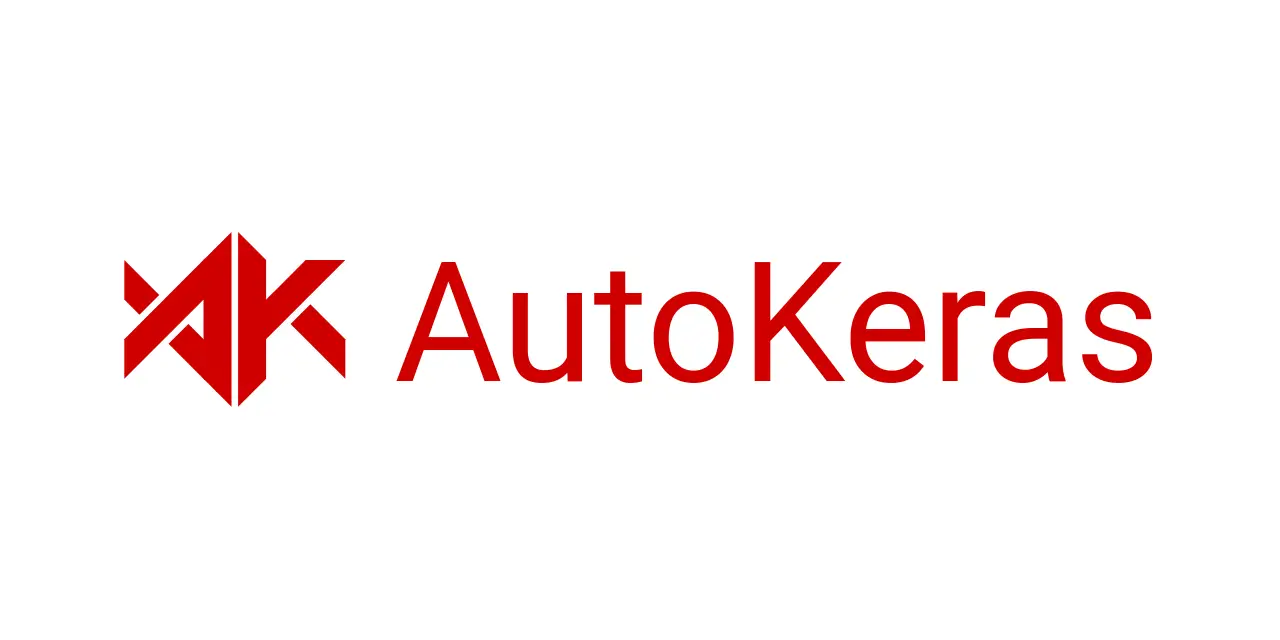
2. H2OAutoML
H2OAutoML is one of the most popular AutoML tools. H2O has an industry-leading AutoML functionality that automatically goes through every algorithm and their hyperparameters to deliver a leaderboard of the best models. This platform has been utilized by over 18k organizations around the world and is very famous in both the R & Python communities.
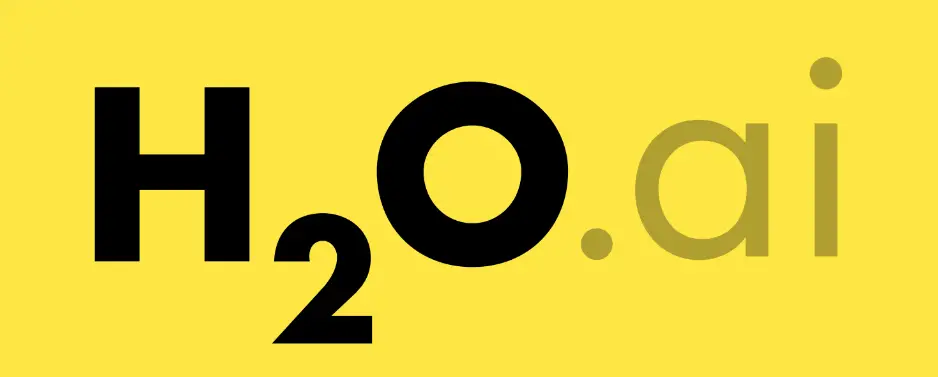
3. JADBio AutoML
JADBio AutoML is one of the excellent AutoML tools to avail user-friendly ML without coding. Beginners in data science, researchers, etc., can utilize this AutoML software to start with ML and interact with the machine learning models effectively. There are only five steps to utilize AutoML; first, preparation of the data for analysis, performing predictive analysis, discovering knowledge, interpretation of the result, and application of the trained machine learning model.
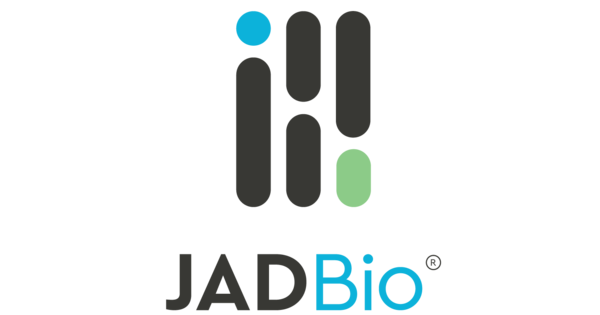
4. Google Cloud AutoML
Google Cloud AutoML is one of the great, well-known, and top AutoML software to prepare custom machine learning models with limited ML expertise or skill as the business need might arise. Cloud AutoML offers simple, protected, and flexible products with an easy to utilize & understand graphical interface.
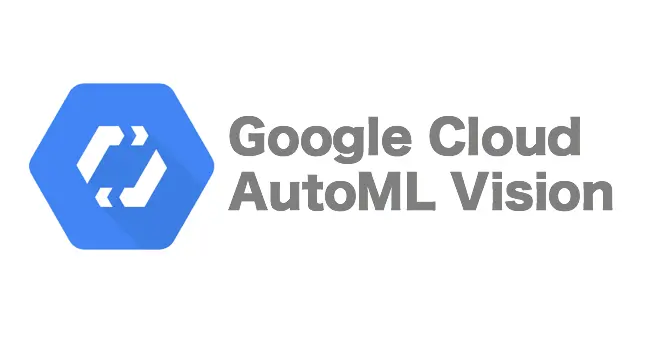
5. MLBox
MLBox is great AutoML software and Python library with numerous helpful features, such as fast reading & distributed data pre-processing or formatting, highly powerful feature selection & leak detection, accurate hyperparameter optimization in high-dimensional space, state-of-the-art predictive models for classification & regression, and prediction with models’ interpretation.
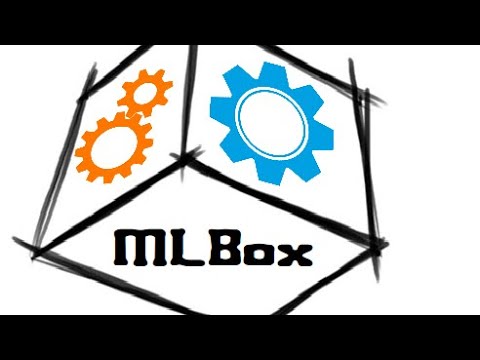
6. MLJAR
MLJAR is one of the top-rated AutoML software. Its AutoML framework gives a state-of-the-art performance. MLJAR AutoML software utilizes advanced feature engineering, ensemble building and model stacking to build accurate machine learning systems, all in an automated manner.
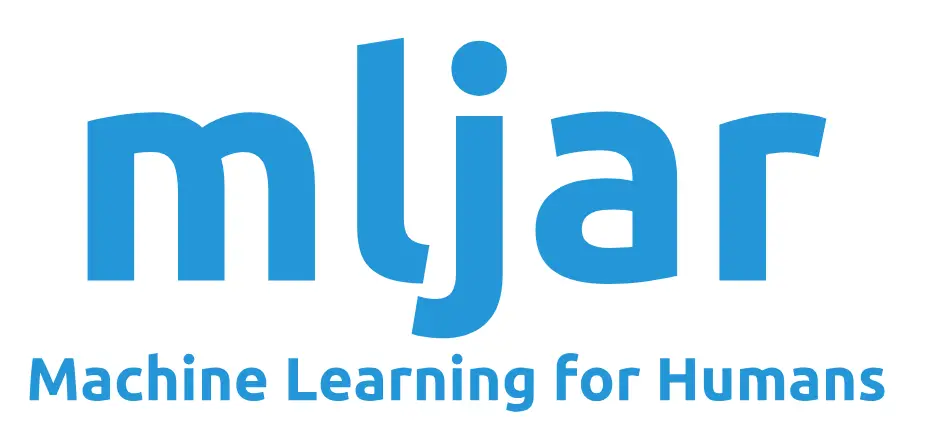
7. Azure Machine Learning
Azure Machine Learning is very well-known AutoML software to build and deploy ML models with Microsoft Azure. This AutoML tool plays its role to boost ML model creation with the automated ML no-code UI or SDK. It enables both professional and non-professional data scientists by automating more time-consuming and tedious tasks of model development.
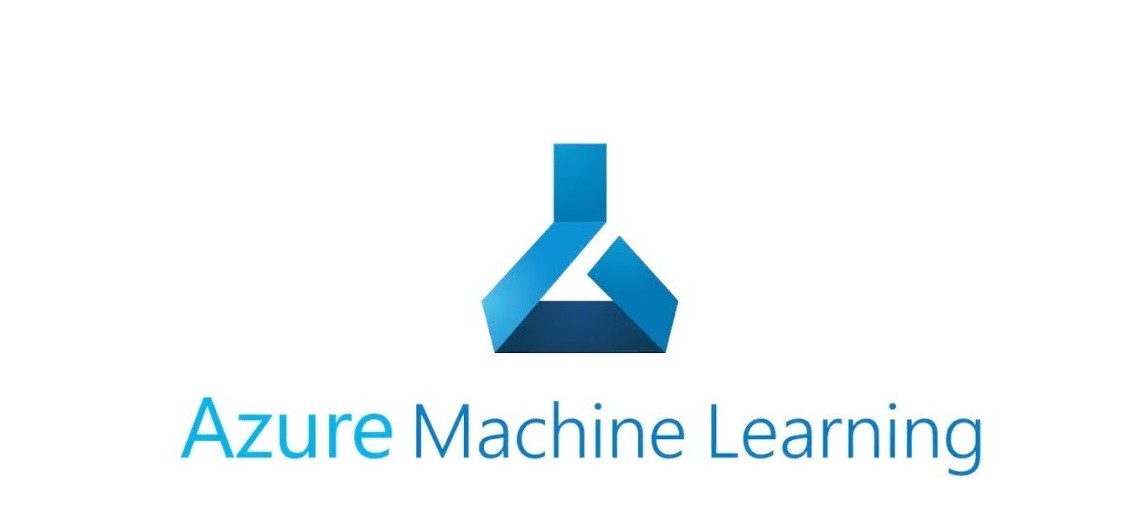
8. Enhencer
It is an AutoML Platform that mainly focuses on transparency & practicality. The best-in-class user interface permits you to construct machine learning models in just a couple of clicks. Enhencer offers easy-to-understand performance metrics that simplify model tuning and evaluation. Its interfaces also permit you to track the model’s performance.
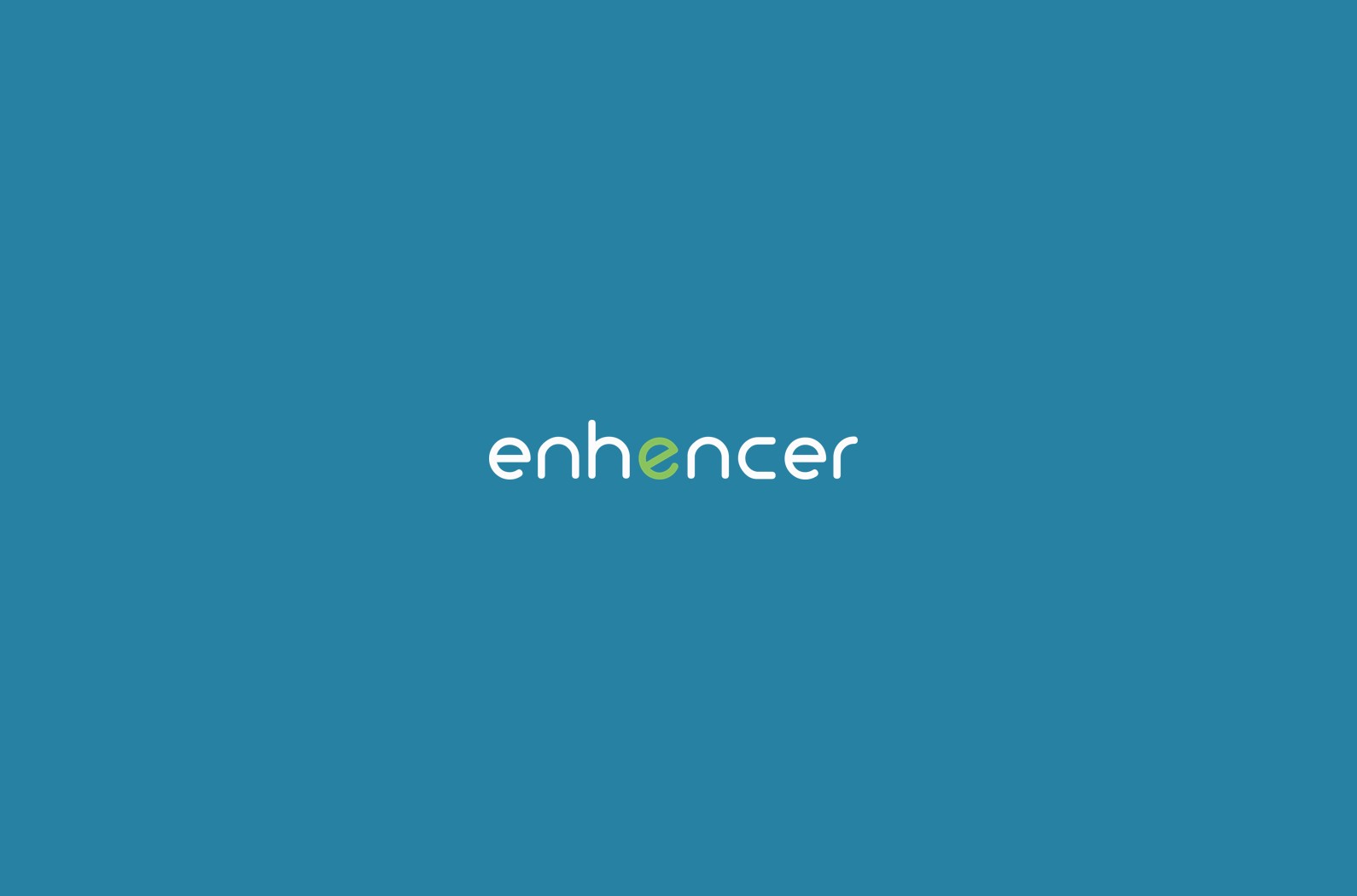
9. Tazi.ai
Tazi’s AutoML is understandable continuous ML from data and humans empower business domain experts to utilize machine learning to make predictions and take necessary actions. It uses several ML models with supervised, semi-supervised, unsupervised algorithms, learning altogether and combined dynamically.
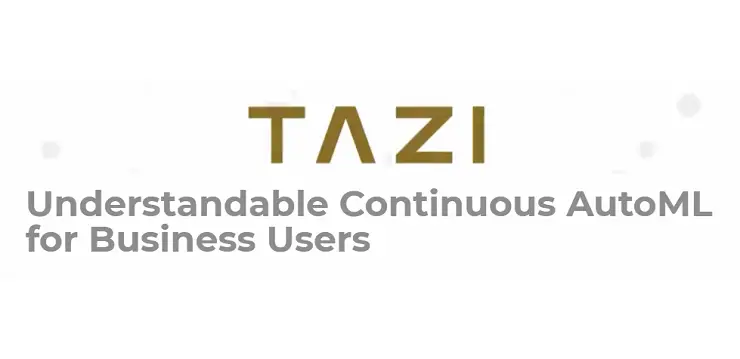
10. SMAC
Sequential model-based algorithm configuration is abbreviated as SMAC. It is a flexible tool for optimizing algorithm parameters. SMAC is extremely effective for hyperparameter optimization of ML algorithms, scaling better to high dimensions and discrete input dimensions in comparison with other algorithms.
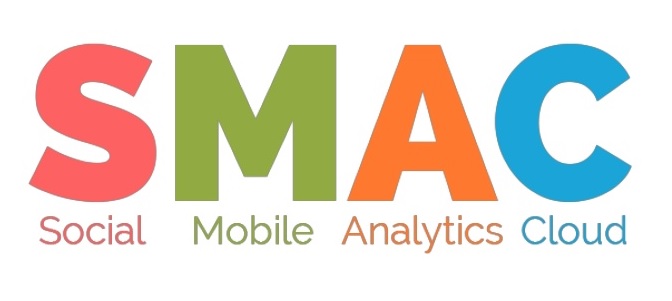