In all conceivable contexts, the epidemic has altered the trajectory of existence by enabling digitalization. Privacy-enhancing technologies (PETs) have taken the lead in these times. One of the most promising and practical solutions to address and lessen the problems brought on by technology itself is the PET group of technologies. Privacy is unquestionably a crucial requirement, especially when the number of users’ personal information on the network keeps growing daily. Let’s learn more about some of the most popular privacy-enhancing technologies (PETs) in 2022.
1. Differential privacy
Differential privacy prevents the disclosure of any personal data. By adding a “statistical noise” layer to the dataset, this cryptographic approach makes it possible to explain patterns of groups within the dataset while protecting individuals’ privacy.
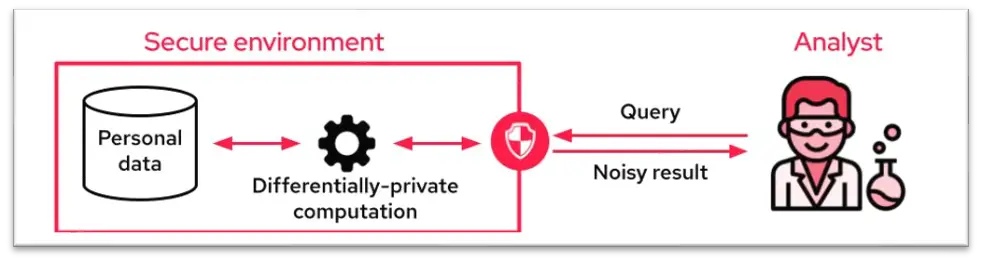
2. Homomorphic Encryption
Homomorphic encryption is often called the “holy grail” of encryption methods. The ability to compute in ciphertext or encrypted form is the most intriguing aspect of homomorphic encryption. Homomorphic encryption was traditionally viewed primarily from a computationally intensive perspective. Homomorphic encryption is now among the best privacy-enhancing technologies available.
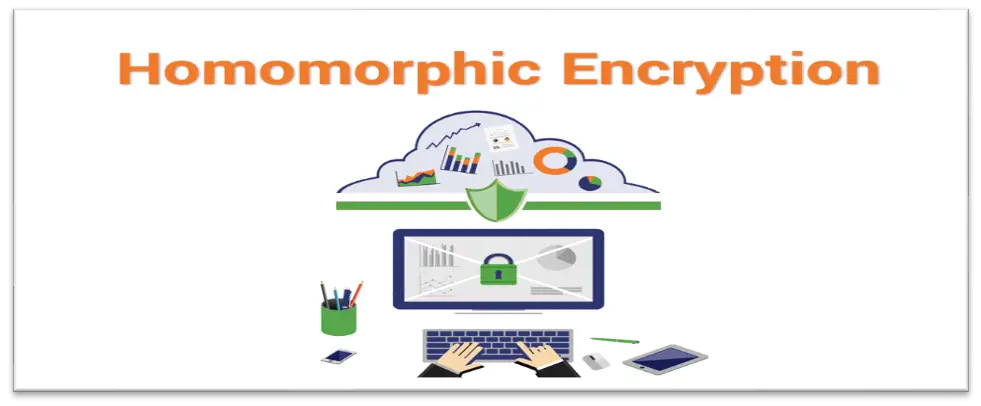
3. Secure Multi-Party Computation (SMPC)
A distributed computing system or technology called Secure multi-party computation (SMPC) expands the ability to compute values according to requirements. It is a branch of homophobic encryption, with the main distinction being that SMPC enables users to input data from several encrypted data sources. It is feasible to use machine learning models in SMPC because they can handle enormous volumes of data. The most typical instance of secure multi-party computation is secret sharing.
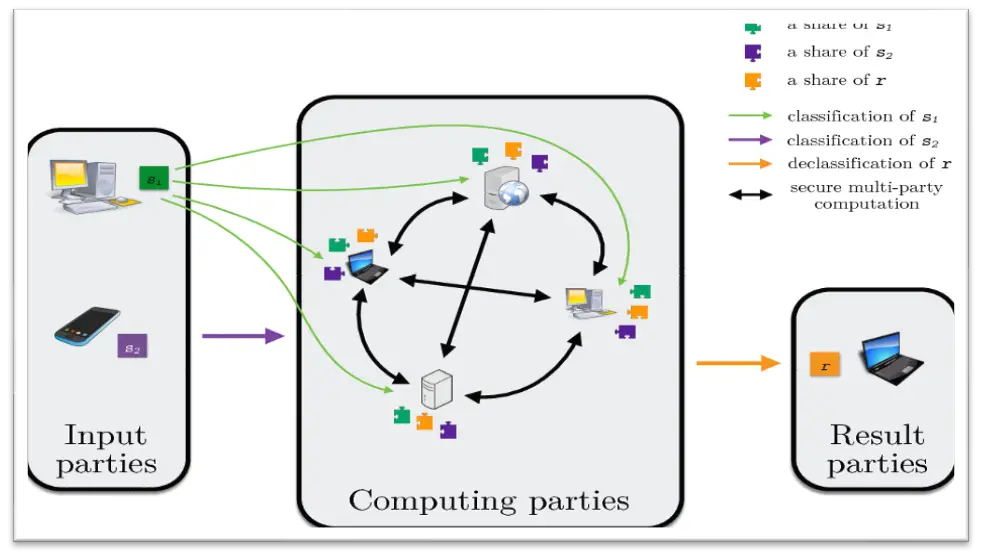
4. Generative Adversarial Networks
In reality, Generative Adversarial Networks, or GANs, are an artificial intelligence subtype that specializes in producing algorithms in pairs. Unsupervised machine learning is an everyday use for generative adversarial networks. Their use incorporates a system for providing extraordinarily effective modeling of original data that pits two neural networks against one another. Synthetic data sets are the most common application of GANs.
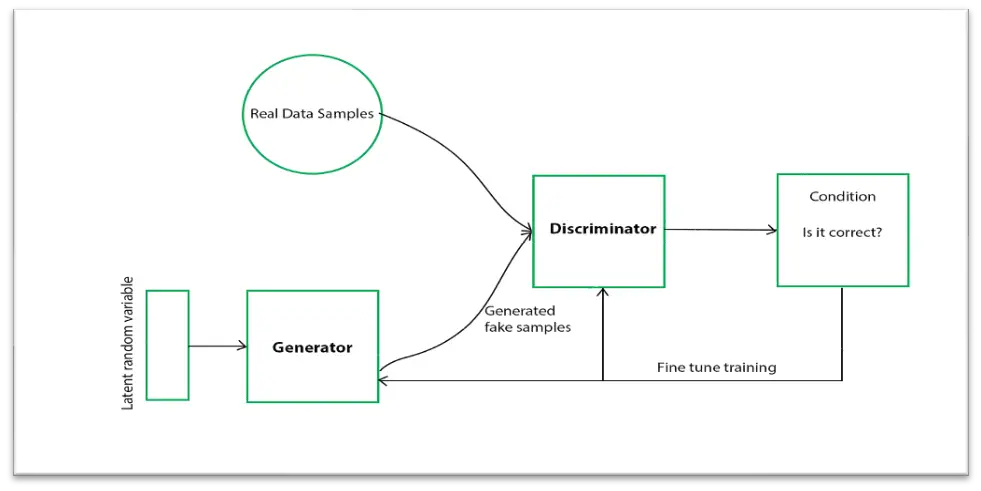
5. Zero Knowledge Proof
Organizations frequently mention zero-knowledge proofing as one of the best privacy-enhancing technologies. With the help of cryptographic methods, this technology enables data confirmation without revealing the underlying data that supports it. It improves a party’s capacity to demonstrate that they know another party. The prior party need not provide the latter with further information, which adds to the convenience.
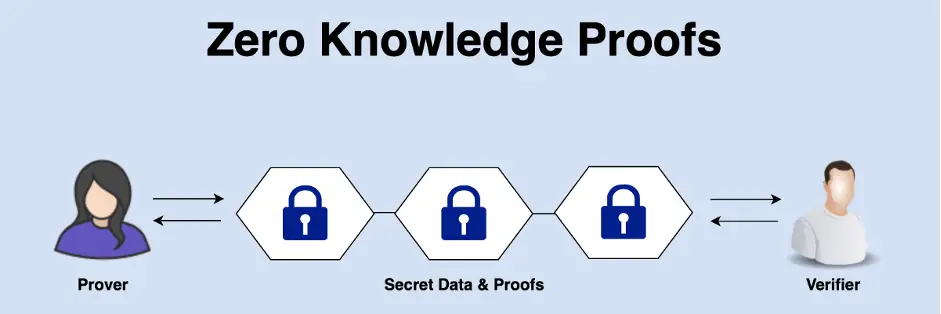
6. Synthetic Data Generation
Synthetic data has been artificially created using various algorithms, including ML If you’re interested in privacy-friendly technology, you need to transform the data into a test environment accessible to third-party users need to generate synthetic data with the same statistical properties.
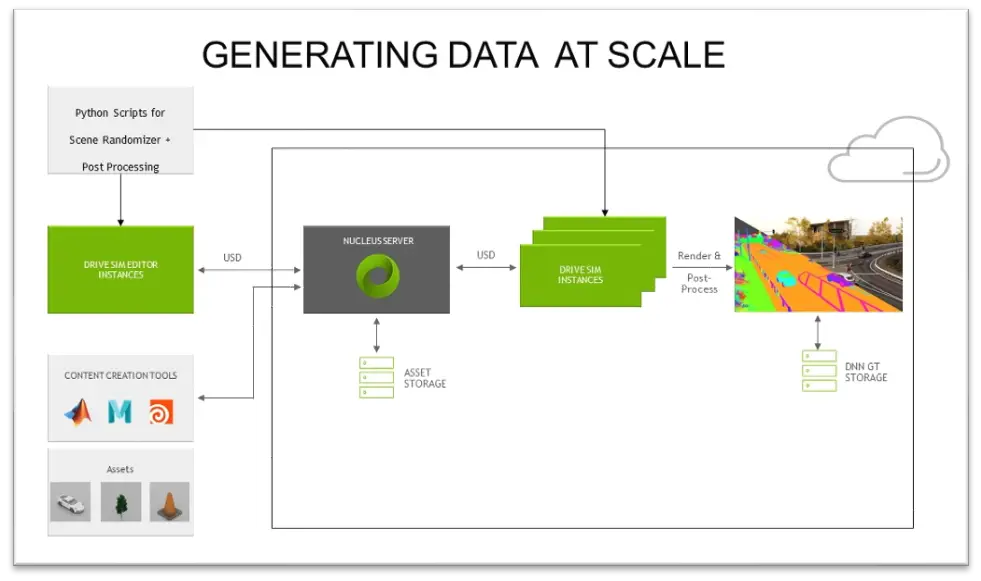
7. Identity Management
Identity management solutions use various platforms, such as distributed ledger technology and local processing, to help individuals authenticate their Identity management solutions can also leverage machine learning at the device level for verification and authentication. Thus, people without internet access develop secure connections and exchange identity-based credentials without the intervention of centralized intermediaries.
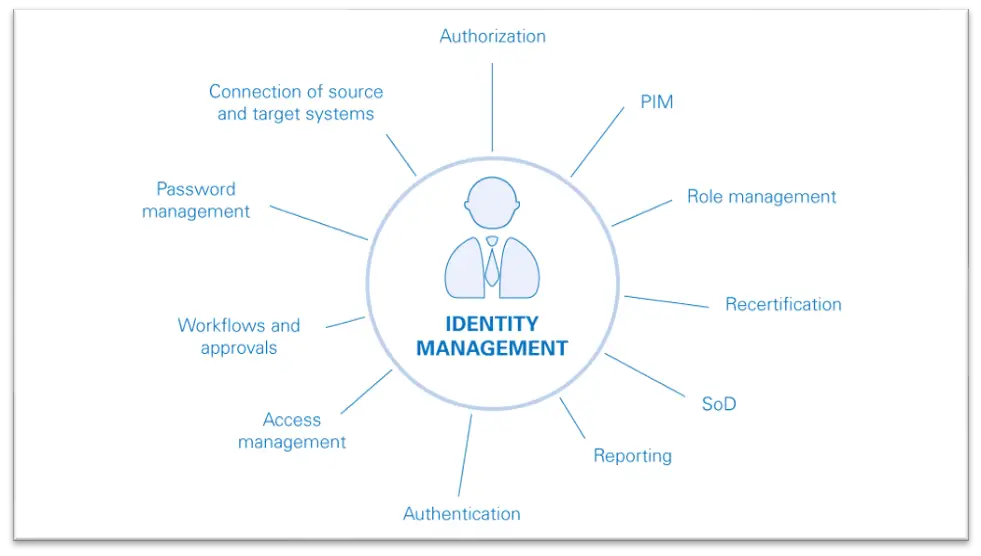
8. Federated Learning
Compared to other PETs, federated learning stands out because it trains or activates algorithms on different devices and servers with data assets without requiring any Data is not scattered or shared anywhere other than where it originated. With rapid server fragmentation, users can also use it to reduce data by significantly reducing the amount of data kept in cloud storage. It has significant application potential in IoT use cases.
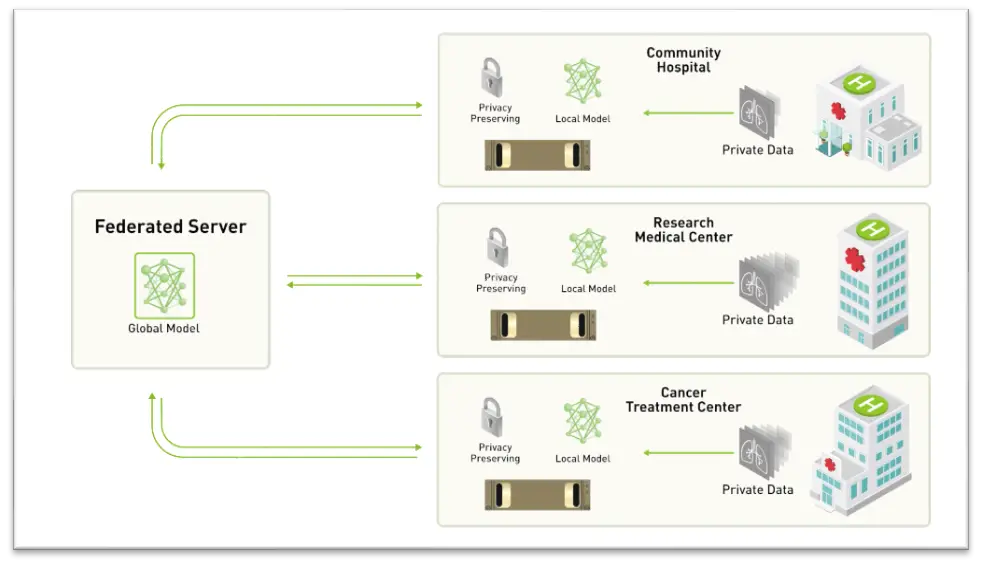
9. Pseudo Anonymization
Pseudo-anonymity is a form of concealing an individual’s identity by replacing field values with The pseudo-anonymization process deletes only part of the identity data. Shuffling and data masking are two methods of pseudo-anonymization, which mix the original values with masked letters.
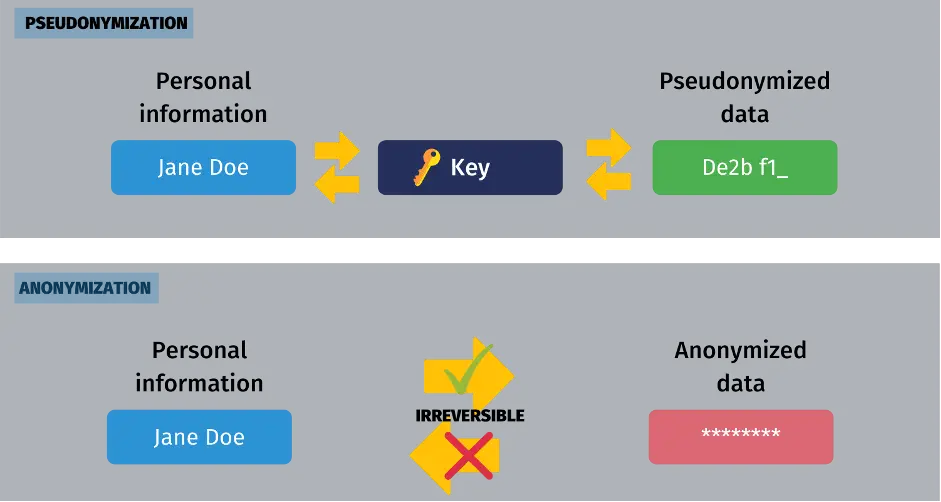
10. Edge Computing And Local Processing
The combined use of edge computing and local processing is also one of the best privacy-enhancing technologies. That involves leaking applications, data, and services from centralized nodes at the network end. That is a clear highlight in case the device needs high speed or doesn’t have any constant connection. Through local processing, service providers can reduce the amount of data they have to collect or store centralized or in the cloud.
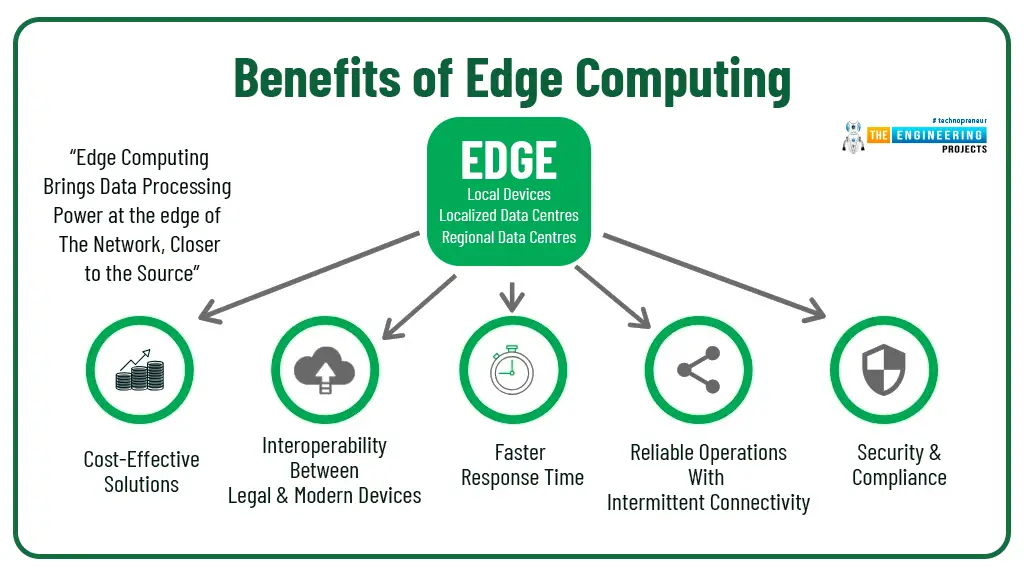